article
Master Data Management: Laying the Groundwork for AI-powered Health Data Management
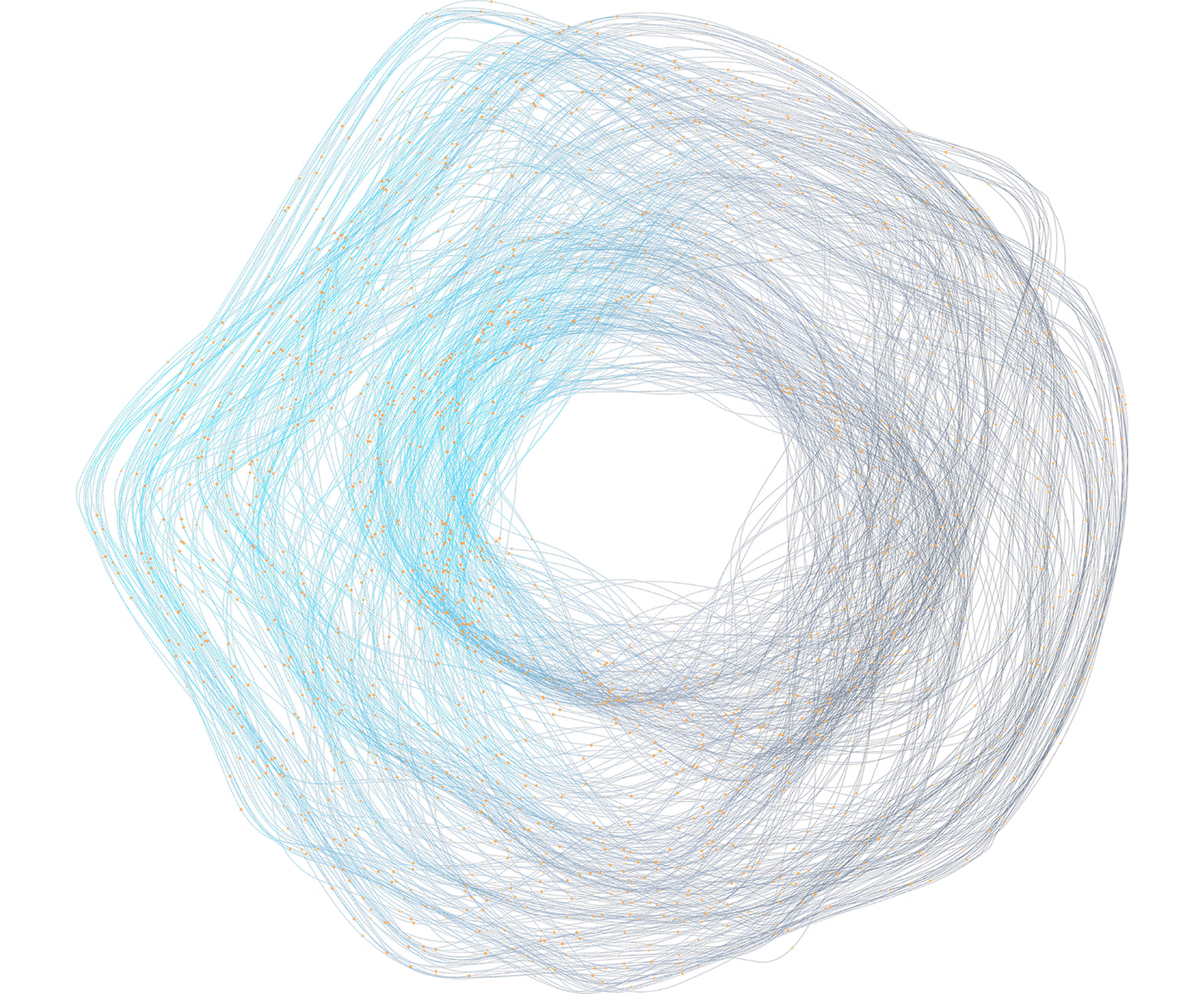
SECTIONS
No technology has been as transformative across industries as artificial intelligence. It’s revolutionized how organizations manage and leverage data, offering advanced analytics capabilities at unprecedented speed and scale. It’s not surprising, then, that healthcare executives often jump right to AI when taking on digital transformation.
But in the race to adopt AI, many healthcare enterprises fail to achieve clean, accurate, well-integrated data to support it, let alone replace legacy systems to ensure that their data management systems can effectively leverage AI capabilities.
A recent study by the National Institute of Health (NIH) on current barriers to AI integration in healthcare summed this problem up aptly, citing data quality as a primary barrier to successful AI adoption.
“It is difficult to acquire and amalgamate large, high-quality data sets that are complete and diverse,” researchers stated. “AI platforms are limited by the concept of ‘what goes in is what comes out,’ implying that the algorithm is only as good as the data source ‘teaching’ it.”
Master data management (MDM) on the right health data management platform is the key to bridging this critical gap. By establishing a single and authoritative source of truth, MDM mitigates the risk of data discrepancies and misguided insights, laying the foundation for AI to reach full transformative potential.
Quick Takeaways:
- MDM-supported data fabric architecture is the gold standard and lays the foundation for AI to deliver its full value potential.
- By implementing MDM within an HDMP, organizations can achieve greater data consistency, quality, interoperability, compliance, governance, and security.
- In turn, AI can operate using complete and accurate datasets that drive valuable, usable insights.
- Important AI capabilities supported by MDM include robust analysis across datasets, predictive analytics, real-time insights, and proactive security measures.
Master Data Management and Your HDMP: Understanding the Connection
Demand for seamless interoperability in healthcare has driven the adoption of health data management platforms (HDMPs) to centralize and manage data across entire ecosystems. MDM-supported data fabric architectures are emerging as the gold standard within these platforms, providing the unified, flexible framework required to analyze and exchange data effectively.
With MDM and a data fabric in place, organizations can achieve:
- Data Consistency/Standardization: Standardized data formats and terminologies that ensures patient identifiers, provider details, and clinical codes are uniform across all systems
- Data Quality Assurance: Clean, validated data free of duplicates, inconsistencies, and errors
- Interoperability and Data Integration: Data that’s seamlessly integrated and exchanged across sources and ecosystems customized to each consuming application’s data needs to drive ubiquitous data strategies
- Regulatory Compliance: Data management practices that can be easily aligned with current regulatory rules and standards
- Data Governance: Clear policies and procedures for data management, such as access controls and data stewardship processes, to ensure accountability and transparency
- Enhanced Decision-Making: Single source of data truth to drive informed decisions based on accurate and up-to-date information
- Operational Efficiency: Streamlined processes such as patient admissions, billing, and reporting that reduce administrative burdens and improve workflow efficiency
In these ways, MDM is more than an add-on—it’s a foundational component of HDMPs that ensures the data that lives there is both reliable and useful.
The impact of MDM can be seen across healthcare settings. In hospitals and health systems, it ensures consistent patient information across departments, from emergency care to outpatient services. For payers, MDM standardizes member data, facilitates accurate claims processing, and streamlines policy administration. In life sciences settings, MDM supports the integration of clinical trial data to power efficient research and development.
These are just a few examples, but they underscore the central role MDM plays in creating the unified and reliable data environment necessary to implement advanced technologies like AI.
Laying the Foundation for Optimized AI and Analytics
When organizations jump too quickly to AI, analytics, or other data-driven applications without focusing on establishing MDM processes and data fabric to support it, the resulting solution is hampered by inconsistent, inaccurate, and fragmented data that can lead to unreliable analyses and misguided insights.
But when the right groundwork is laid for AI to perform at its full potential, there are a myriad of ways it can enhance outcomes from both a clinical and operational perspective.
AI-Driven Insights from Unified Patient Data
AI systems excel at identifying patterns and making predictions based on comprehensive datasets. With MDM standardizing patient, provider, member, and other identifiers and consolidating data from various sources, AI can access a fully unified, longitudinal view of a patient’s medical history to deliver these insights. Comprehensive and unified datasets enable AI tools to conduct more accurate analyses, such as predicting disease progression or identifying risk factors for chronic conditions.
Leveraging Integrated Data for Advanced AI Models
The power of AI is amplified when it can analyze a wide array of data types, including clinical records, genomic information, imaging data, lab results, and even external social determinants of health. MDM facilitates the integration of these diverse datasets, providing AI with a rich source of information.
For example, AI can analyze integrated clinical and genomic data to identify genetic predispositions to certain diseases, allowing for early detection and intervention. The broad dataset enables AI to develop more sophisticated models that consider many aspects of patient health, leading to better diagnostic and treatment outcomes.
Optimizing Operational Efficiency through Predictive AI
AI’s predictive capabilities can significantly enhance operational efficiency in healthcare settings when the data it analyzes is accurate and complete. By analyzing both historical and real-time data, AI tools can forecast things like patient admissions, staffing needs, and resource allocation requirements, enabling organizations to prepare appropriately and avoid shortages or overages.
Gaine Coperor Health Data Management Platform (HDMP): The Best of Both Worlds
Gaine Technology solutions are designed specifically to address the unique challenges of healthcare and life sciences industries. We understand the timely need to adopt AI into your data strategy and to have a repository of cleansed, accurate, de-identified, deduplicated, and consistent data for all of your data needs. With Coperor, you can lay the right foundation for sophisticated digital transformation without sacrificing speed of deployment. Even more importantly, you can maintain an agile data strategy that can align with changing technology advancements, regulatory standards, and patient demands.
Learn more about how to transform with Coperor today.