article
The Power of Common Data Models in Healthcare: Bridging the Gap in Data Management
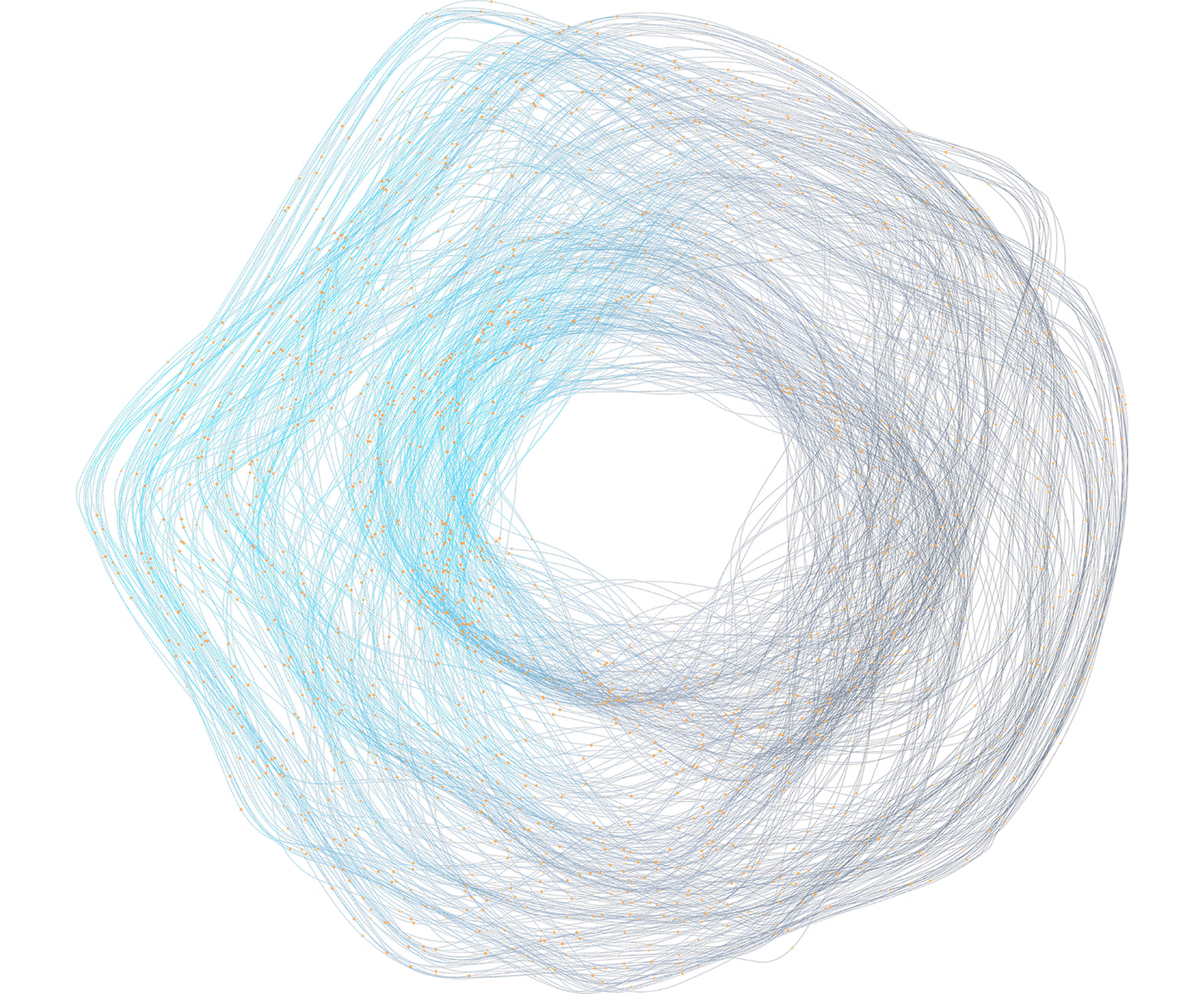
SECTIONS
Unifying Data Strategies for Enhanced Healthcare Outcomes
Within the healthcare and life science industries over the years, there has been a struggle to identify how best to model data for its intended purpose. The term “Common Data Model” or CDM has hit the lexicon of the industry, but few people understand what that really means, let alone how to construct and implement such a concept.
Put simply, a common data model (CDM) is a standardized framework for organizing and representing medical data from diverse sources in a consistent format. Across healthcare and life sciences, it provides a shared data language and structure that allows different healthcare systems, applications, and researchers to more easily exchange, integrate, and analyze data.
Key aspects of common data models in healthcare include:
- Standardized data structure and formats for representing clinical concepts like patients, diagnoses, procedures, medications, etc.
- Consistent terminology and coding systems across datasets
- Defined relationships between different data elements
- Metadata and semantic definitions to ensure common understanding of the data
Common data models are used in healthcare to:
- Facilitate data sharing and interoperability between different healthcare organizations and systems
- Enable large-scale observational research and analytics across multiple data sources
- Support regulatory decision-making and post-market safety surveillance of medical products
- Streamline the development of analytics and applications that can work across multiple datasets
- Consistently measure and report on quality metrics across different organizations
- Leverage claims data to support revenue cycle processes
- And many other uses
Important CDM Considerations
Balancing Standardization and Flexibility: The Challenge of Industry-Specific CDMs
A common data model, in general, is standardized, potentially subject-area specific, and usually can accommodate extensions based on specific use cases or customizations. The idea of a common data model also means that the metadata and associated definitions of the attribution can be universally adopted across enterprise solutions. More times than not, this has proven to be a huge struggle. Industry-specific common data models, especially in healthcare and life sciences, need to be very specific and functionally relevant to the type of organization trying to use one. Even within healthcare, insurance payers will usually need a different common data model than, let’s say, a healthcare delivery system because of the nuances and functional needs.
This diversity in requirements highlights the need for adaptable CDMs that can cater to various healthcare sectors while maintaining a core structure. For instance, a hospital system might prioritize patient care data and clinical outcomes, a payer might prioritize organizing their provider information for more accurate provider directories, while a pharmaceutical company may focus more on drug efficacy and safety data. Despite these differences, a well-designed CDM should provide a foundation that allows for customization without sacrificing interoperability.
Cross-Domain Relationships: The Key to Unlocking Data’s Full Potential
A crucial aspect of an effective common data model is the ability to connect and navigate data across different domains, rather than keeping data in isolated silos. We call these connections “cross-domain relationships.” These relationships are as important as the data itself and should be treated as such within the model. If a common data model doesn’t support managing and maintaining these relationships, it will fail to meet many important use cases and business needs, ultimately leading to unsuccessful outcomes. This means being able to properly relate areas such as patient, members, providers, organizations, locations, facilities and many more to transactional data like claims and clinical information, so that the necessary context of information can be extrapolated and interpreted correctly.
The importance of these cross-domain relationships cannot be overstated. They enable healthcare organizations to gain a holistic view of their data, facilitating more comprehensive analyses and insights. For example, linking patient demographic data with clinical outcomes and treatment protocols can reveal patterns that inform personalized medicine approaches or population health strategies.
Beyond Structure: Ensuring Data Integrity and Governance in CDMs
A common data model (CDM) is designed to support operational, reporting, and analytical systems across an organization. However, to be truly effective, a CDM must be complemented by robust data management capabilities. These capabilities ensure that critical aspects such as data quality, validation, lineage, and history are properly maintained. It’s important to note that merely implementing a CDM doesn’t guarantee that all downstream users are working with consistent information. Without proper data management, different teams or systems may interpret or use the data inconsistently, potentially leading to conflicting results when answering similar questions. This inconsistency can undermine confidence in the data and the insights derived from it. Therefore, comprehensive data management is essential to maximize the value and reliability of a CDM.
Implementing robust data governance practices is crucial to ensure the integrity and reliability of the CDM. This includes establishing clear data ownership, implementing data quality checks, and maintaining comprehensive metadata. By doing so, organizations can build trust in their data assets and promote consistent use across different departments and systems.
The Gaine Coperor Health Data Exchange Model
Defining a common data model is not easy nor for the faint of heart and starting from scratch usually means drawn out requirements gathering, development, implementation, and testing cycles − usually still resulting in incomplete outcomes. Within Gaine, we’ve spent nearly 20 years developing out the common data model used by our proprietary Coperor Health Data Management Platform (HDMP). Referred to as the HDX (Health Data Exchange) model, we’ve looked at dozens of systems, use cases, and industry standards in developing this model. We’ve also spent years battle testing this model in various environments across in some of the nation’s largest payer, provider, and life sciences organizations to ensure we have a robust model that is also extensible, since we inevitably will run into new use cases needing refinement or additions to this model.
The HDX model represents a culmination of industry best practices and real-world applications. It addresses common challenges in healthcare data management, such as integrating disparate data sources, maintaining data quality, and supporting evolving regulatory requirements. By leveraging pre-built components and standardized structures, the HDX model significantly reduces the time and resources required to implement a comprehensive CDM.
While compatible with interoperability standards like HL7 FHIR, HDX is over five times larger and more granular. With HDX, FHIR is a simple subsets of what you get out of an HDMP with the added benefit of basing all that information being interconnected, tracked over time, and based on a foundation of MDM capabilities to ensure that data is cleansed, deduplicated enriched with reference data, and consolidated into longitudinal profiles of patients, providers, members, claims, and other domains.
The HDX model is in support of the Gaine Health Data Management platform and helps organizations accelerate the time to value of a CDM implementation. Organizations may also want to fully adopt our CDM for their own internal needs and get the benefit of time and research we’ve done to accelerate success for their own initiatives. The long-term benefit of utilizing our platform/model or adopting it as your own is being hardened for future changes, acquisitions, migrations, or business use cases.
Rather than building one from scratch that may have to be altered or rewritten over short periods of time, this approach gives the foundational building blocks to be adaptable to the future of healthcare. As the healthcare landscape continues to evolve, with increasing emphasis on value-based care, precision medicine, and population health management, the need for robust and flexible CDMs will only grow. By adopting a proven model like HDX, healthcare organizations can position themselves to meet current challenges and future opportunities, ensuring they remain at the forefront of data-driven healthcare innovation.
Contact us to learn more about how Gaine can accelerate your data-driven healthcare initiatives with a mature, robust healthcare common data model.