blog
Data Quality Management in Healthcare: The Complete Guide
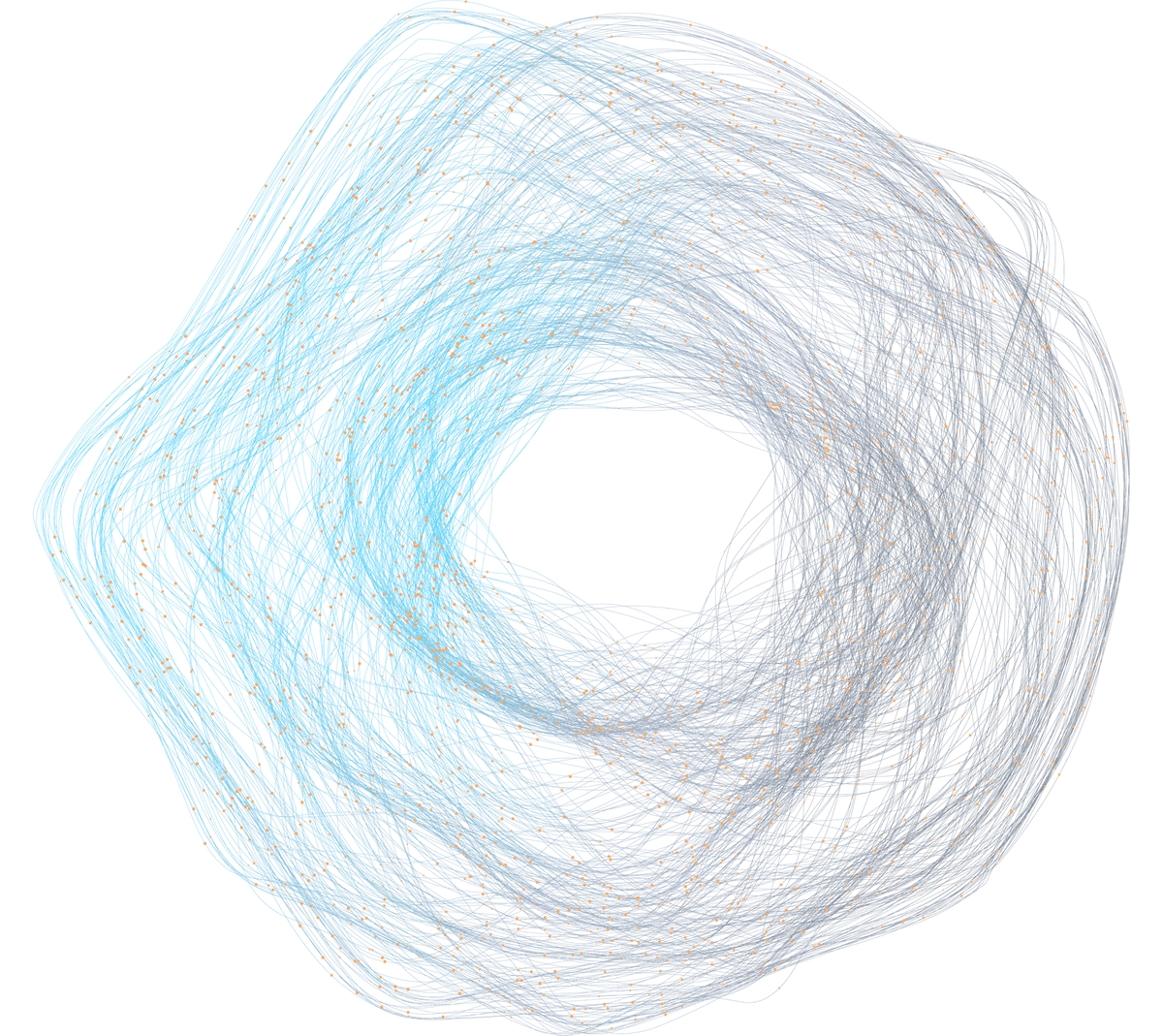
SECTIONS
In a healthcare industry that’s becoming increasingly reliant on data, effective data quality management has become a critical part of every organization’s strategy for success. From making the right patient care decisions to optimizing operational efficiency, data quality management in healthcare is fast becoming a make-or-break capability.
In this guide, we’ll walk you through what exactly is meant by data quality in general, the reasons it has become so important in healthcare, and 10 best practices for leveling up your data quality management to achieve the outcomes you envision.
Key Takeaways:
- There are six primary factors determining data quality: accuracy, timeliness, consistency, validity, uniqueness, and completeness.
- Data quality management in healthcare is especially paramount because it can impact patients’ safety and healthcare outcomes.
- For healthcare organizations, data quality can also impact regulatory compliance and research and development success.
- Best practices for effective data quality management in healthcare include strong governance, regular audits, standardization, data-driven culture, and master data management (MDM).
Data Quality Defined
Data quality refers to the condition of data and how well it meets its intended purpose. It uses six criteria to measure this:
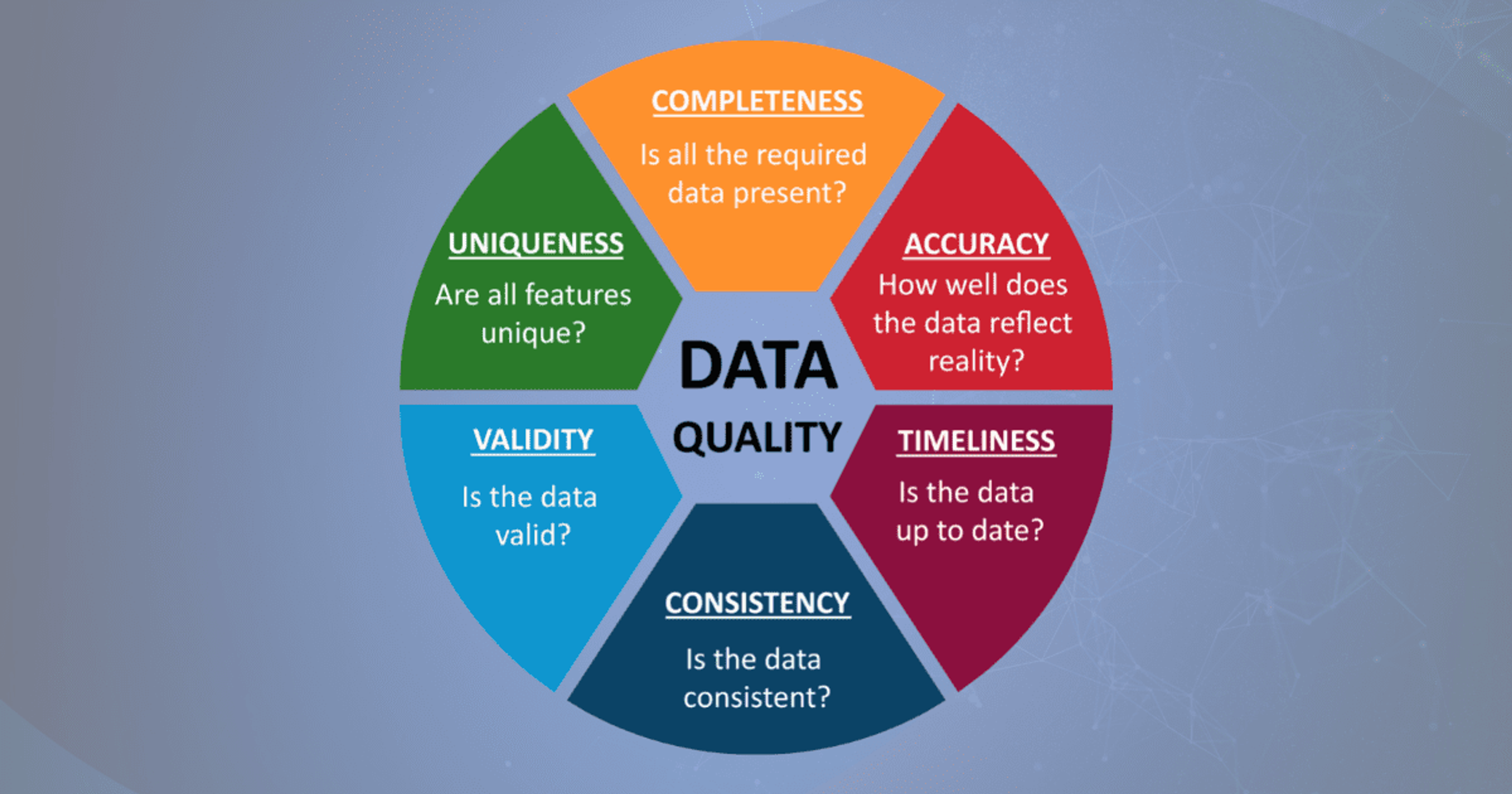
Image Source
- Accuracy: This pertains to whether the data correctly represents the real-world event or item it aims to capture. Accurate data is free from errors and has been verified against trusted sources or benchmarks.
- Timeliness: This refers to the age of the data and its relevance to the current context. Timely data is up-to-date and available when needed, ensuring decisions are made based on the most recent information available.
- Consistency: Data consistency ensures that data across all platforms and systems remains uniform. It means that data does not show any discrepancy when compared across different data silos, ensuring a seamless flow of information.
- Validity: Valid data adheres to a specific format, range, pattern, or definition. For instance, a date should be in a recognized date format, and a patient’s ID should match the predefined structure.
- Uniqueness: This criterion ensures that data entities are recorded once and only once, preventing duplications. For instance, a patient should have a single unique record, eliminating any redundancies and confusion.
- Completeness: Complete data is comprehensive and lacks no essential details. In the context of healthcare, this might mean that a patient’s medical history is fully recorded, without any gaps or omissions.
Ensuring that data meets these requirements should be a fundamental part of every company’s data management strategy, especially organizations in the healthcare industry.
Why Data Quality in Healthcare is Paramount
The importance of data quality in healthcare is even more paramount than in many other industries—it can be a determiner of critical patient safety factors, like care quality and regulatory compliance. At the same time, it also has business implications for healthcare organizations related to operational and cost efficiencies. Let’s take a closer look.
Patient Safety
At the heart of healthcare lies the safety and well-being of patients. Erroneous patient data can lead to misdiagnoses, inappropriate treatments, or overlooked medical conditions. Ensuring data quality is not just a procedural necessity—it can be a matter of life and death.
Regulatory Compliance
Healthcare organizations operate within a web of regulatory requirements. Accurate data is critical to adhere to these regulations, avoid penalties, and ensure that patient rights, especially concerning data privacy, are upheld.
Operational Efficiency
High-quality data allows healthcare organizations to streamline operations, allocate resources effectively, and reduce redundancies. This ensures that patients receive timely and efficient care, and organizations can operate at optimal capacity.
Financial Integrity
Inaccurate data can lead to financial issues such as billing errors, insurance claims rejections, and other discrepancies. Ensuring data quality safeguards the financial health of healthcare institutions and ensures financial decision makers are informed by accurate information.
Research and Development
The healthcare industry heavily relies on research and data-driven innovations. Quality data is pivotal for successful clinical trials, drug development, and other research endeavors, ensuring that research findings are valid and can be trusted.
Decision Making
From administrative decisions about hospital operations to clinical decisions about patient care, quality data provides the solid foundation healthcare organizations need. Without it, decisions are often based on guesswork or flawed information, leading to subpar outcomes.
As healthcare continues its shift towards digitization and data-driven operations, the imperatives of data quality management in healthcare will become even more pronounced. In the next section, we’ll delve deeper into how healthcare organizations can ensure and maintain this data quality, driving excellence in every facet of operations and care provision.
10 Best Practices for Data Quality Management in Healthcare
Data quality management in healthcare requires an active strategy that prioritizes strong oversight, uses consistent standards and protocols, fosters a data-driven culture, and uses the right tools to enhance it. Let’s look at 10 best practices that you can implement to uplevel your data quality management strategy.
1. Establish Clear Data Governance
Implement a robust data governance framework that defines user roles, responsibilities, standards, and processes to ensure that data quality is maintained throughout the organization. Strong data governance has a three-pronged benefit: It protects people, prioritizes equity in healthcare, and promotes value.
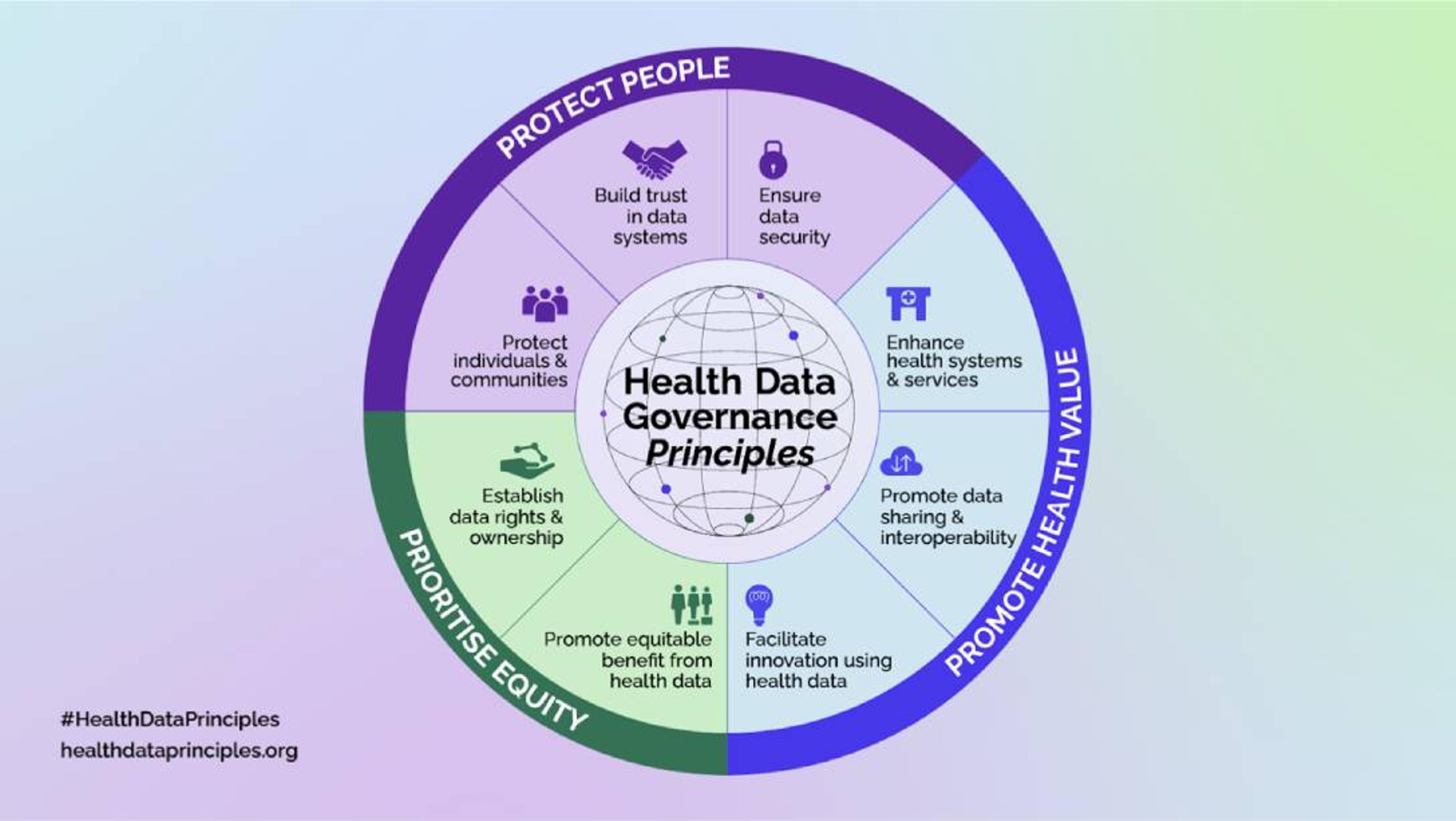
Image Source
2. Regular Data Audits
Periodically review and audit your data sources. These frequent data quality checks help to identify inconsistencies, errors, and redundancies. Implement corrective measures based on audit findings.
3. Data Validation Checks
Set up automated validation rules to ensure that incoming data adheres to predefined formats and standards. For instance, ensure that patient IDs follow a specific pattern or that all date entries have a consistent format.
4. Training and Education
Continuously educate and train staff about the importance of data quality and the procedures required to ensure it. This includes learning best practices for data entry, understanding potential sources for data errors, and knowing the right steps to rectify them.
5. Use Standardized Data Entry Protocols
Implement standardized protocols for data entry to reduce variability and inconsistencies. This could involve using dropdown menus, predefined formats, or automated data capture tools.
6. Implement Data Quality Tools
Invest in advanced data quality tools that can detect, correct, and prevent data issues. These tools can often integrate with existing systems, ensuring continuous data quality management.
7. Foster a Culture of Data Quality
Make data quality a part of organizational culture. When every member of the team understands and values the importance of high-quality data, it becomes an inherent aspect of daily operations.
8. Data Quality Dashboards
Create dashboards that provide real-time insights into important data quality metrics. This enables quick identification of areas that need attention and facilitates proactive data quality management.
9. Feedback Loops
Establish feedback mechanisms where end users can report data issues they experience. This both helps in timely resolution and also identifies potential areas for improvement.
10. Leverage Master Data Management
Master data management (MDM) solutions allow organizations to create a single, consistent view of data from multiple sources, ensuring uniformity and reducing duplication. Adopt an MDM platform solution to centralize your most important data and maintain optimal quality at all times.
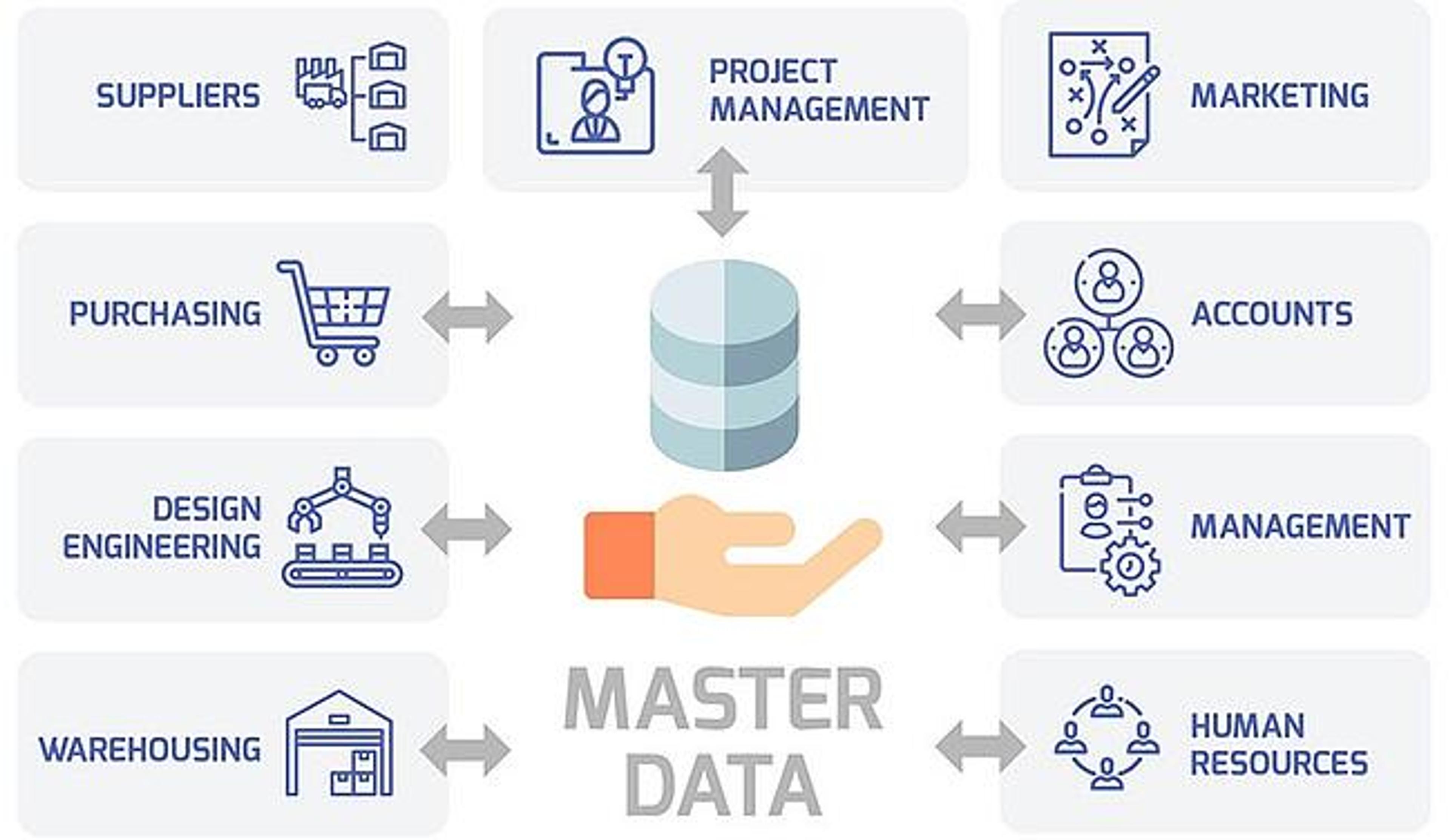
Image Source
By adopting these best practices, healthcare organizations can ensure that they not only maintain but continuously improve data quality. Given the critical nature of most healthcare decisions, these practices become foundational in delivering exceptional patient care and optimizing operational efficiencies.
Remember, in healthcare, data quality isn’t just a technical requirement—it’s an ethical obligation.
Gaine MDM Solutions
Learn more about how Gaine’s ecosystem-wide MDM solutions can help your healthcare organization achieve better data management outcomes today.