blog
Building the Ideal Data Governance Framework
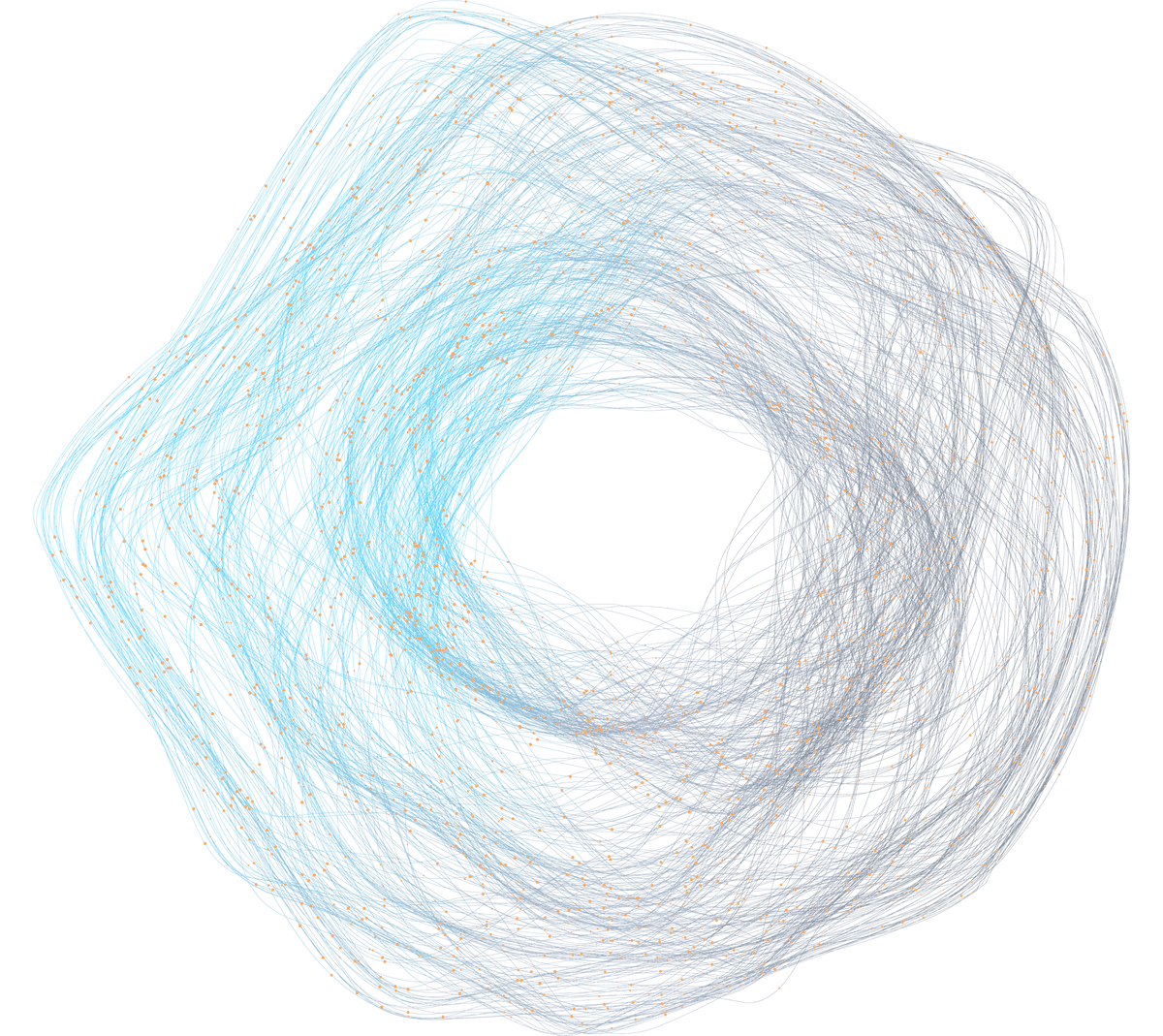
SECTIONS
The healthcare industry deems data management and governance critical for operational efficiency, patient safety, and regulatory compliance. However, establishing an effective data governance framework is challenging, and many organizations fail in the process. This guide explains what data governance is and outlines four steps to build a robust data governance framework in your healthcare organization.
Key Takeaways
- Data governance involves managing data assets to ensure their quality, consistency, security, and usability.
- Poor data quality can cost organizations 20-30% of total revenue, emphasizing the need for data governance.
- Following the four steps outlined in this guide, organizations can establish a strong data governance framework.
What Is Data Governance?
Data governance refers to the systematic management of data assets within an organization to ensure their:
- Quality
- Consistency
- Security
- Usability
It is a blend of policies, standards, processes, and technologies that coordinate to control and optimize data throughout its lifecycle. Establishing accountability and responsibilities among key stakeholders is the foundation of an effective data governance framework. An effective data governance program will ensure data handling by authorized aligns with both organizational objectives and regulatory compliance.
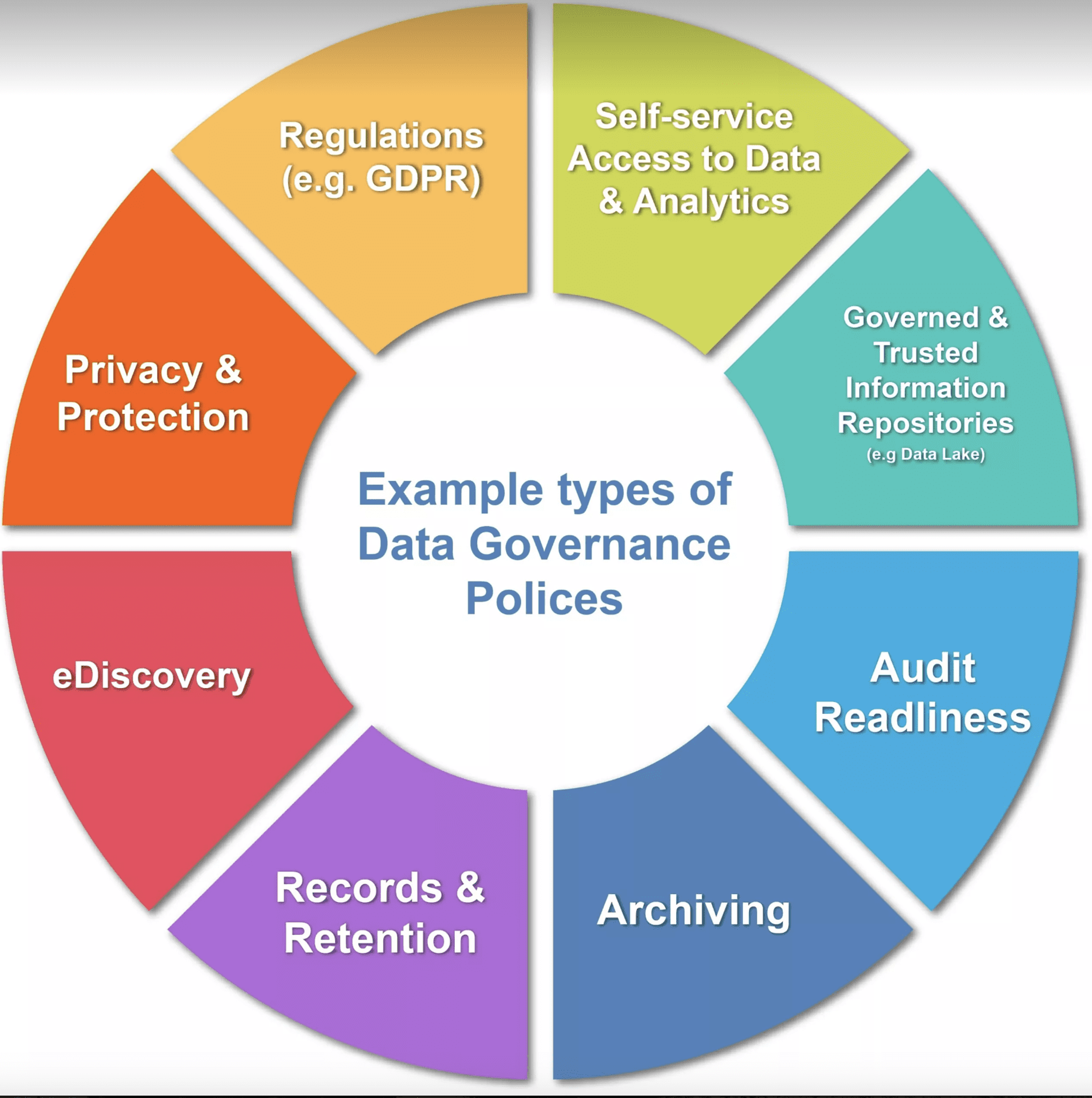
Image Source: Mentortechgroup.com
To accomplish this, data governance programs typically contain these eight kinds of policies:
- Self-service data analytics
- Repositories and data lakes
- Audit readiness
- Archiving
- Records and retention
- eDiscovery or compliance with Freedom of Information Act (FOI) requests
- Privacy and protection
- Regulations and compliance
With a focus on these critical areas, data governance ensures data quality management and the continuous monitoring and improvement of data accuracy, completeness, and reliability. Consequently, it provides decision-makers with the most pertinent and dependable information for strategic planning and operations.
Data governance employs robust access controls, encryption, and data anonymization techniques to safeguard sensitive information and minimize the risk of unauthorized access and data breaches.
Additionally, data governance promotes the standardization of data elements across an organization. By ensuring consistency in data definitions and formats, standardization enables seamless integration and interoperability among different systems and datasets, facilitating more informed and efficient decision-making.
Building a Data Governance Framework
Building a data governance framework may seem like a complex task, but the following four steps can help you establish a robust and effective data governance structure in your healthcare organizationOpens in a new tab.
1. Understand Your Data Landscape
The first step to an ideal data governance framework is understanding your data landscape. Knowing what data you have, which systems store what, and how users access it is essential. This includes understanding how much time users spend searching for information.
Recent studies indicate the typical IT worker spends half their day — 4.2 hoursOpens in a new tab on average — searching for existing information. Besides tanking productivity, endless data chasing also greatly contributes to worker dissatisfaction and is a major factor in choosing to leave organizations. By mapping your data landscape and reducing search time, you will improve productivity, enhance decision-making, and improve job satisfaction.
2. Prioritize Data Quality
Many organizations store and even employ faulty or unreliable data because of gaps in their systems and processes. In fact, only 27%Opens in a new tab of data practitioners report fully trusting the data they routinely work with, suggesting a significant portion of data in organizations may lack high quality. Prioritizing data quality is vital to ensure accurate, reliable data supports your decision-making. Practical steps for improving organizational data quality include:
- Establishing data standards: The first step to prioritizing data quality is to establish clear data standards. These standards should benchmark accuracy, completeness, timeliness, consistency, and relevance. This foundation enables users to measure and manage data more effectively.
- Adopting data quality tools: Use advanced data quality tools to continuously monitor and clean data. Analytics platforms can help identify and rectify data issues such as duplicates, inaccuracies, and inconsistencies. These platforms can also automate data cleansing, improving efficiency and reducing human error.
- Promoting a culture of data quality: Lastly, it’s essential to cultivate a culture of data quality within the organization. You must educate employees about the importance of data quality, train them in data management best practices, and encourage them to own the quality of data they handle. Regular training sessions, workshops, and communication campaigns assist greatly in this task.
3. Calculate the Cost of Bad Data
It’s important to understand the cost of bad data. Reports show that bad data quality and efficiency issues cost the average organization 20-30%Opens in a new tab of total revenue. By calculating the cost of poor data quality, you can justify the investment in a data governance framework and build a business case for it.
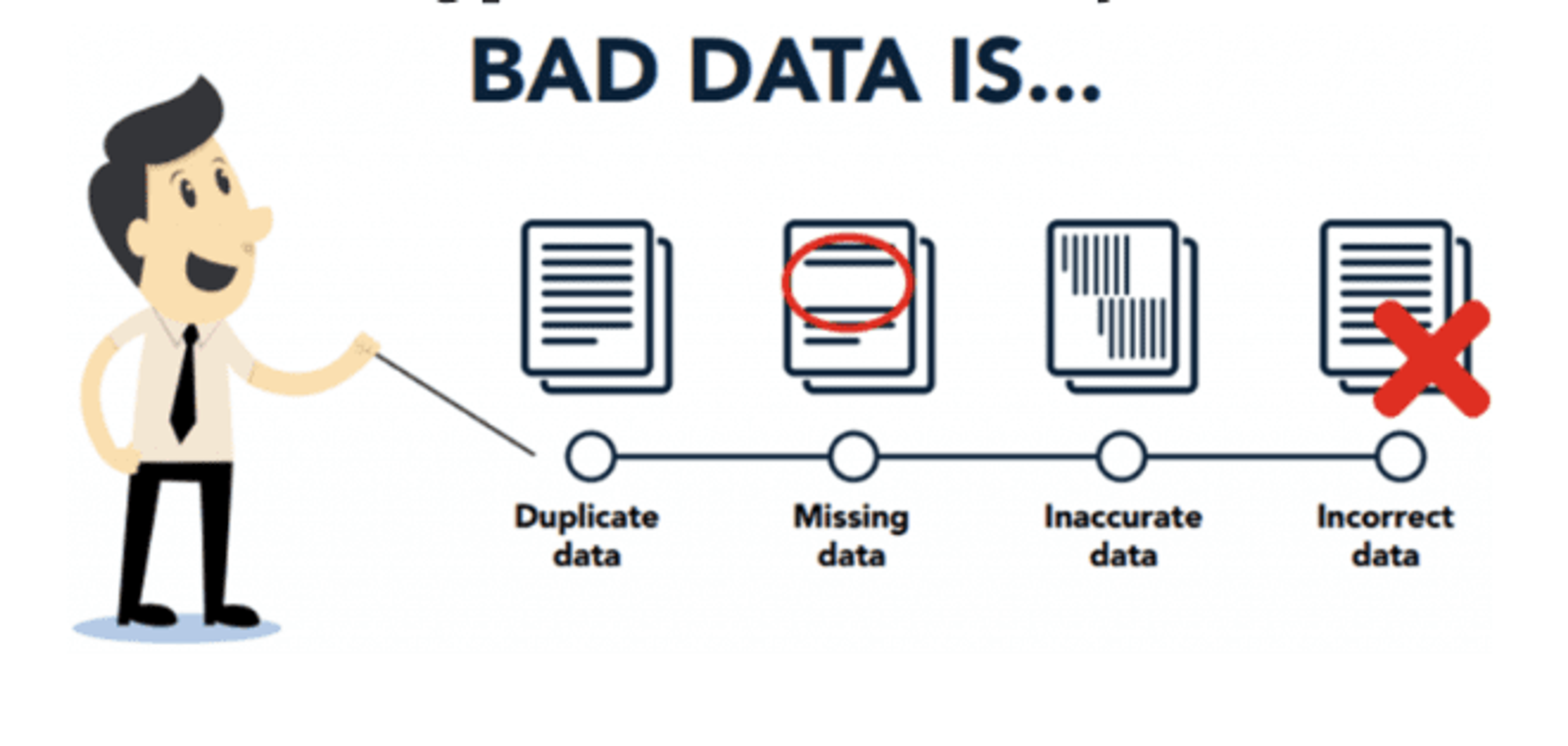
Image Source: ibm.com
Assessing the cost of bad data can be a complex task as it involves considering both direct and indirect costs. Here are two approaches your organization can take:
- Process audits: Start by auditing your existing data-related processes. This includes looking at how often employees need to correct data, how much time they spend on data-related tasks, and how frequently errors occur. You can then calculate the cost of these inefficiencies by considering factors like employee wages and lost productivity.
- Opportunity cost analyses: Consider the opportunities you lose due to bad data. These include lost sales due to inaccurate customer data, missed targeted marketing opportunities, and potential regulatory penalties due to non-compliance. Although these categories are challenging to quantify, industry benchmarks and historical data can still offer you an estimate of potential revenue loss.
4. Leverage Data for Strategic Purposes
Most organizations surprisingly fail to extract value from their data. The average organization never applies 60-73%Opens in a new tab of their stored data to any strategic purpose. Endeavors that connect data to value include using data for:
- Predictive analytics
- Improving patient outcomes in healthcare organizations
- Identifying internal operational inefficiencies
Build Data Governance in Your Organization with the Right Tools and Coperor by Gaine.
In Coperor’s unique healthcare-focused master data management platform, seamless data integration meets unparalleled quality and consistency for improved patient outcomes.
View this demo of Coperor today to learn more.