blog
The Role of Data Quality in AI
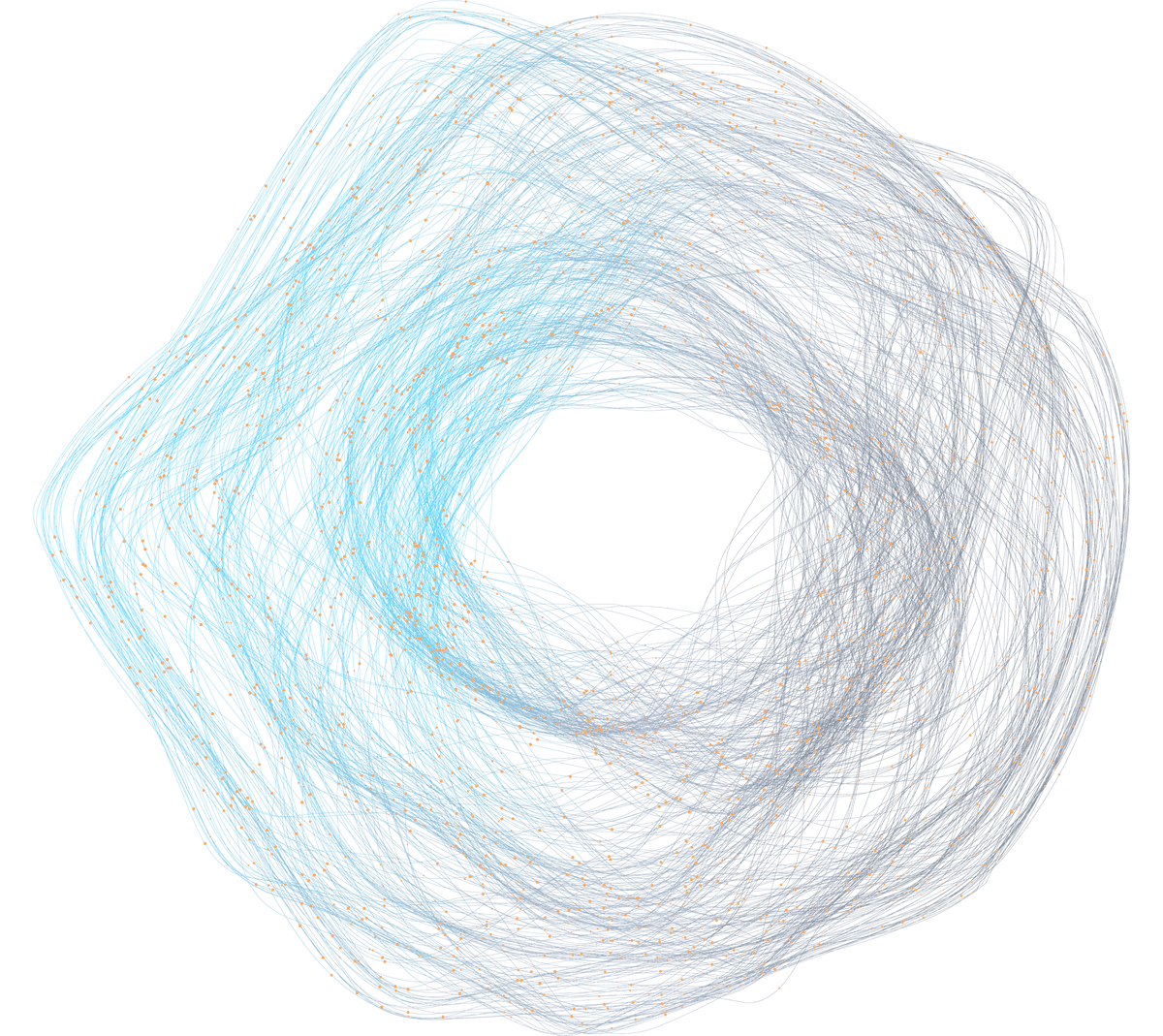
SECTIONS
In today’s evolving technology landscape, artificial intelligence (AI) has become a pivotal asset across healthcare and life sciences industries. However, the effectiveness of these new technologies depends entirely upon data quality in AI systems. Poor training data quality results in inaccurate or misleading outcomes, deviating conclusions, and decision-making processes.
This article explains the role of data quality in AI-based technologies and examines some potential consequences of using AIs trained on poor or incomplete data to make essential business decisions.
Key Takeaways:
- The accuracy and effectiveness of AI technologies heavily depend on the data quality used in their training.
- Poor data quality can lead to misleading outcomes and serious real-world consequences, as the examples Twitter, YouTube, and ChatGPT illustrate.
- Businesses must invest in robust data management systems and establish rigorous data governance frameworks to ensure high-quality AI training data.
The Role of Data Quality in AI
Data quality plays a crucial role in the effectiveness and accuracy of artificial intelligence (AI) systems. AI is only as good as the data developers use to train it, and users of AI systems should not underestimate the importance of ensuring the quality and accuracy of training data.
Training AI with poor data leads to inaccurate results and unreliable insights. For instance, imagine using customer purchase data to train an AI engine to identify repeat customers. If the data used for training is flawed or incomplete, the AI system will make incorrect conclusions. These conclusions have severe consequences, especially in industries like healthcare, where accurate data is vital for decision-making.
Consider an illustration underscoring how AI can mislead if the underlying data qualityOpens in a new tab is poor or improperly formatted. If you trained an AI model using customer purchase data from the table below to identify repeat customers, it would erroneously conclude that females are more frequent repeat customers than males, based on the twice repeated purchases by a female customer named Anne Bond.
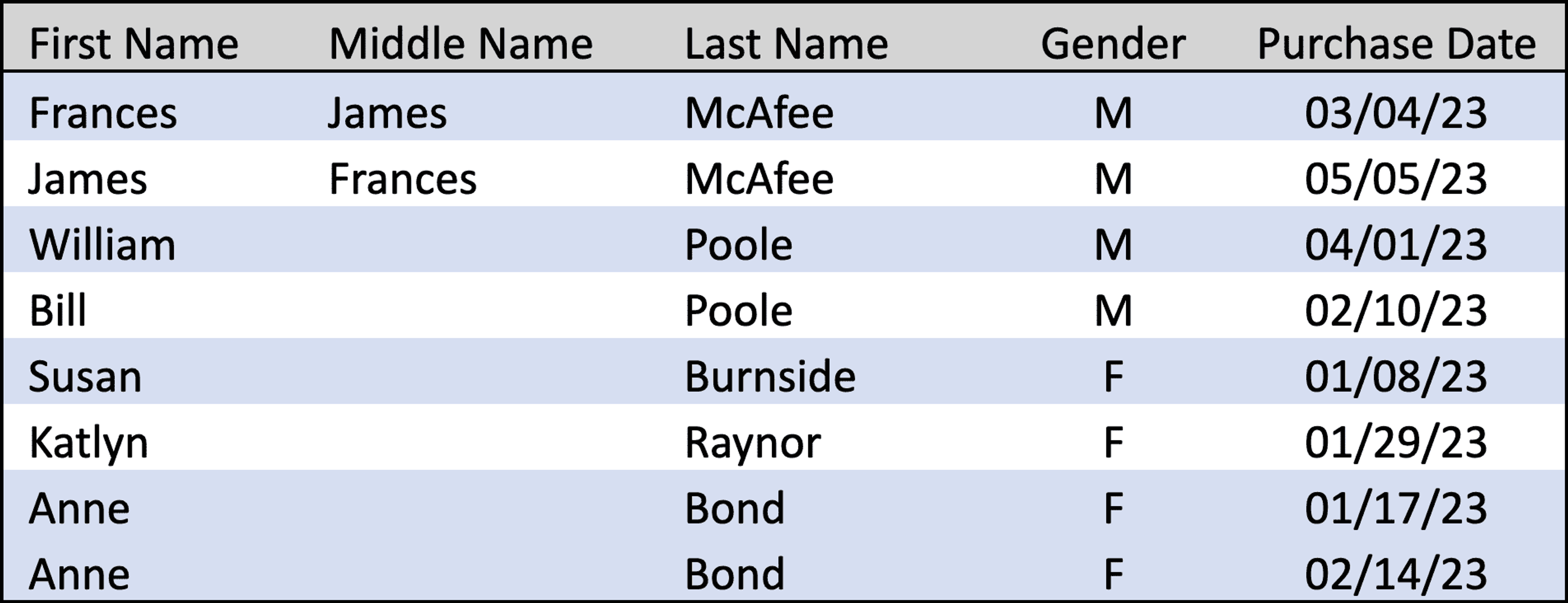
However, after cleansing the data for duplicates and reformatting discrepancies, it’s clear that the table contains variations for the names James Frances McAfee and William Poole. If you correct these discrepancies before training the AI, you get a different outcome. Instead, males are twice as likely to be repeat customers than females, as indicated in the table below.
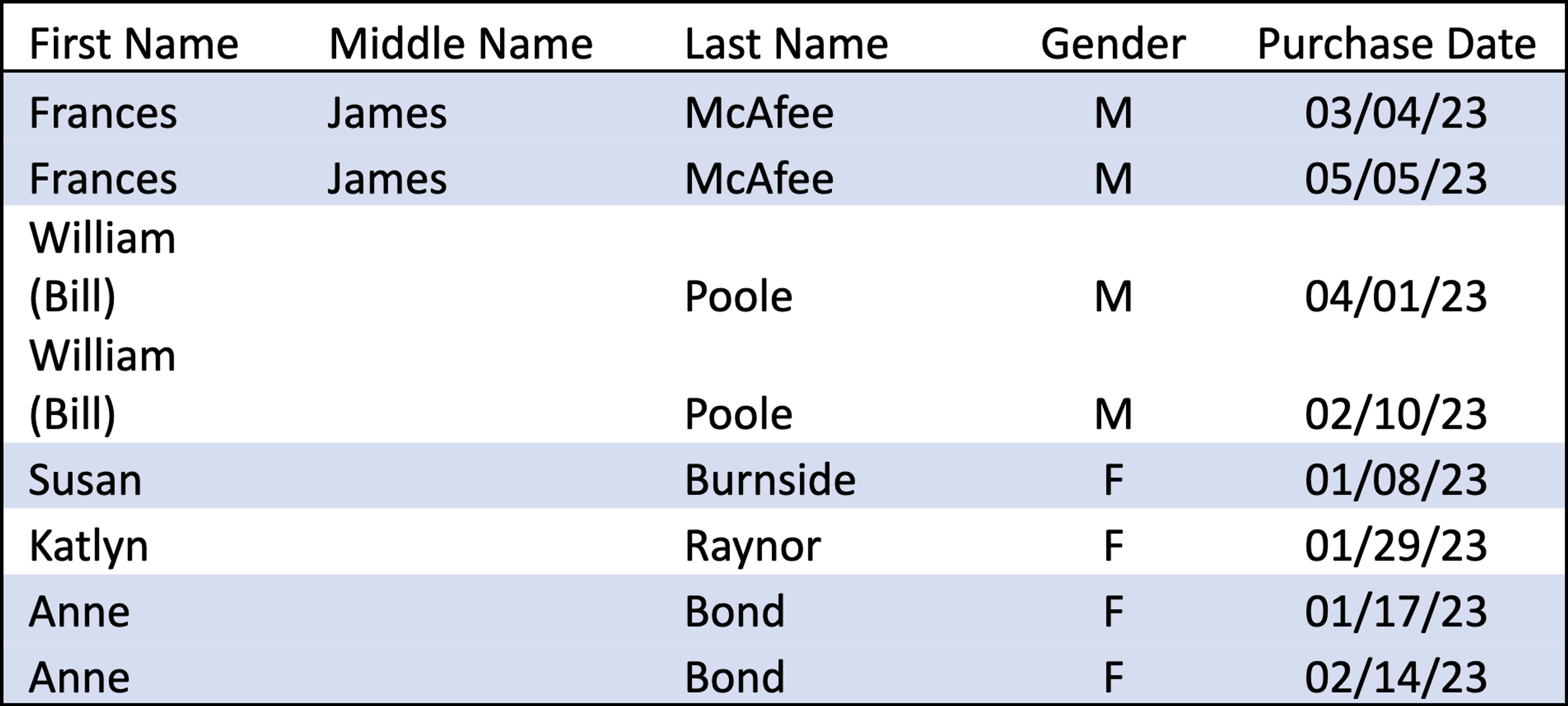
Image Source: Provided by the client.
The disparity in results illustrates on a small scale the indispensable role of data quality in AI modeling. Inaccurate data leads to erroneous conclusions and detrimental effects on business decisions.
Real-World Examples of AI Backfiring Due to Poor Data Quality
The transformative potential of AI is clear. Nevertheless, users must treat it with pragmatism and skepticism regarding undisclosed training data sources. In the last few years, several notable examples of poor training produced unintended consequences. These cases illustrate the pitfalls of unchecked reliance on AI and underscore the need for a balanced perspective on AI’s capabilities and limitations.
1. Twitter Image Cropping Algorithm
In early 2021, engineers for Twitter’s machine learning ethics, transparency, and accountability team disclosed that the platform’s AI image-cropping algorithm exhibited a racial and gender biasOpens in a new tab. Previously, many users had noticed that in previews of images, the algorithm consistently favored showing white individuals over black individuals and men over women. These comments prompted Twitter’s team to evaluate the algorithm and training data for potential biases. In response, Twitter acknowledged the issue and altered the AI, replacing error-prone face detection software based on raw photo data mined from the platform with a computer vision technique called saliency.
2. YouTube’s Content Moderation AI
During the COVID-19 pandemic, YouTube needed to rely heavily on AI for content moderation due to safety concerns for human reviewers. However, the AI mistakenly removed a significant number of videos, as the company had trained it on limited pandemic-related data due to the novelty of the topic and time constraints to implement effective moderation. From April through June, 2020, YouTube’s AI removed over 11 million videos, a rate roughly twice the platform’s average. After reinstating 320,000 videos upon appeal, YouTube changed the AI’s training data and expanded its use of human moderators in AI supervision. This case highlights the shortcomings of an AI system in making nuanced decisions, especially when developers have not used an adequately diverse set of training data scenarios.
3. ChatGPT Hallucinations Cited in Federal Court Case
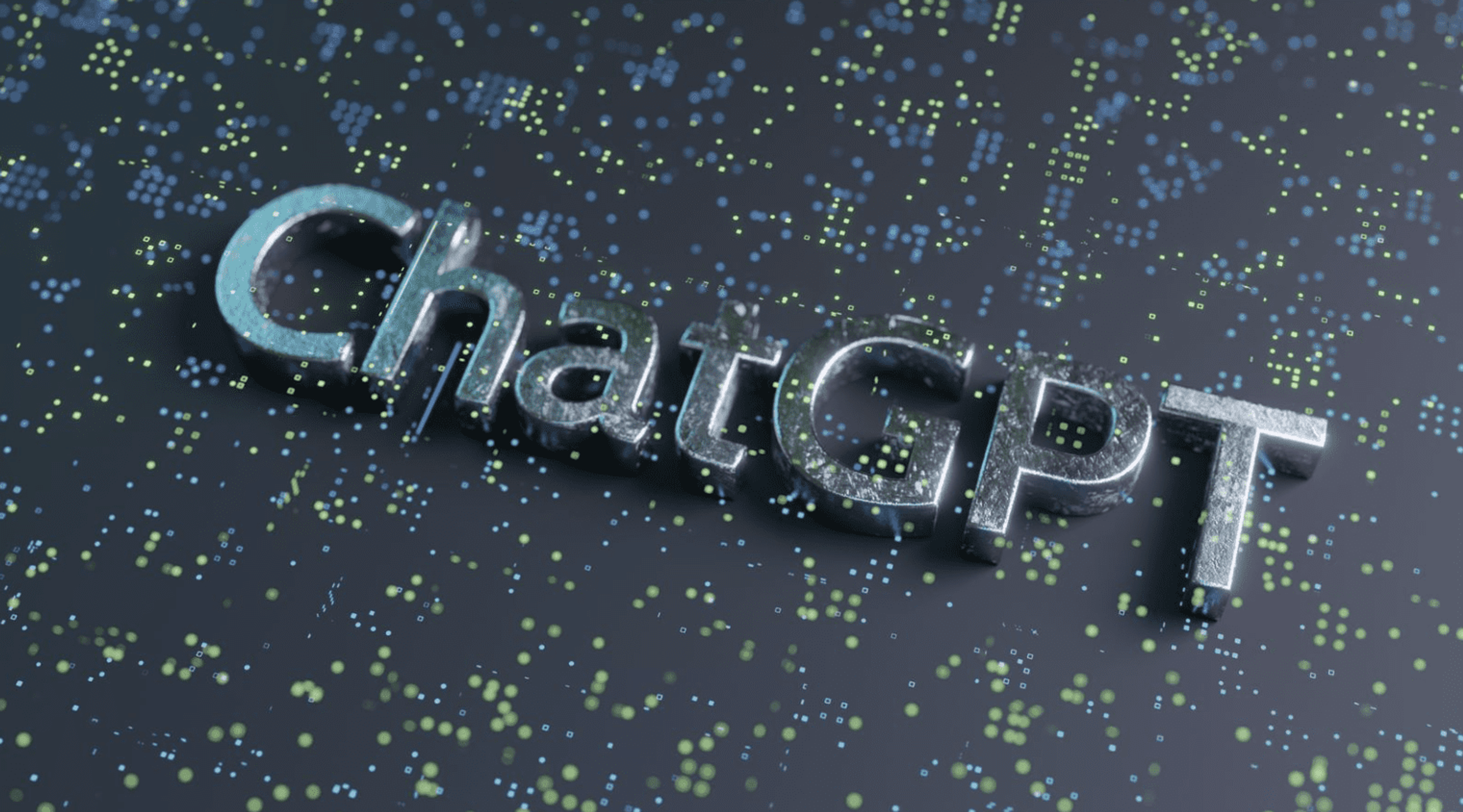
Image Source: LegalDive.com/news/chatgpt-fake-legal-cases-generative-ai-hallucinations
In May this year, a New York lawyer cited multiple fake AI-generated cases in a brief filed in a federal court. Attorney Steven A. Schwartz used OpenAI’s ChatGPT to supplement his legal research for a personal injury lawsuit. However, several of the cases the AI cited were fictitious, leading to possible sanctions against Schwartz and starkly illustrating the importance of data quality and user understanding of their AI tools.
The Way Forward: Ensuring Data Quality
These incidents — among many others — demonstrate that AI outputs are only as good as the data used to train the systems. If the training data contains errors, omissions, or fictitious information, AIs can produce inaccurate or misleading results, often with harmful consequences in the real world. As AI models can’t independently verify the authenticity of their training data or the validity of the outputs they generate, developers are responsible for ensuring high data quality and for users to critically review the AI-generated content before using it to make crucial decisions.
Data quality is the bedrock upon which AI learns and improves. Businesses — especially in healthcare and life sciences, where errors potentially cause patient harm — must invest in robust data management systems that can cleanse, curate, and maintain high-quality data. Establishing rigorous data governance frameworks will also play a pivotal role in guaranteeing the integrity and consistency of the data used for training AI.
Enterprise Master Data Management for Healthcare with Coperor by Gaine
Coperor provides healthcare and life science companies with a master data management platform capable of integrating data across complex tech stacks and networks of contracted partners.
To learn more and view a demo of Coperor in action, visit Gaine today.