blog
Challenges of Data Integration in Healthcare
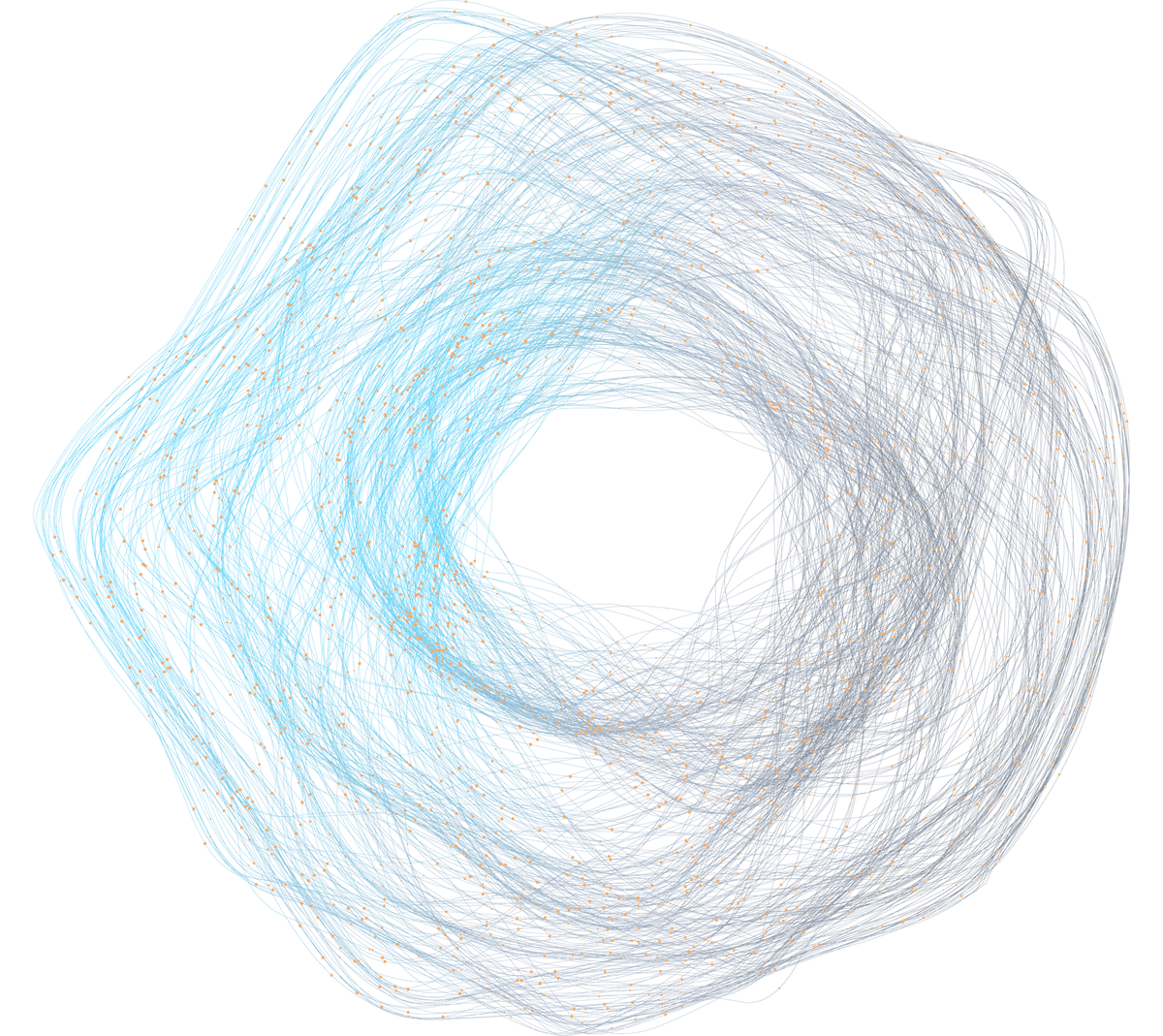
SECTIONS
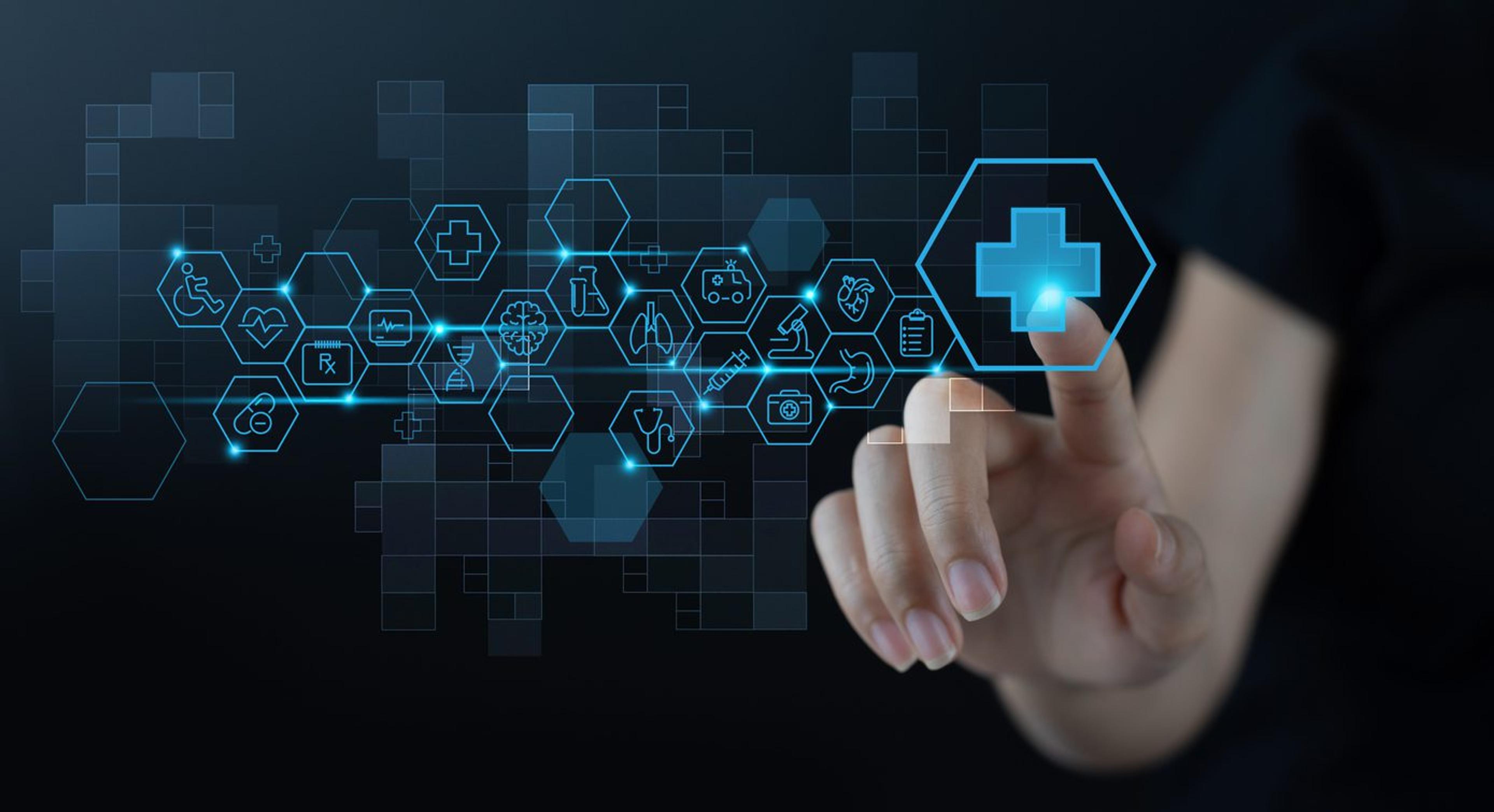
Healthcare data integration is becoming both an increasingly pressing priority and a more complex challenge. In the United States, people see a doctor an average of four times a year. With the U.S. population of 331 million, that translates into at least 132 billion new health records for doctor’s visits alone per year.
Combined with data from pharmacies, insurers, wearable medical devices, and other sources of healthcare data, the total volume of new data points that contribute to a patient’s comprehensive medical history is considerably larger.
Healthcare providers understand that the more they know about a patient, the better decisions they’ll be able to make for immediate and long-term care. Presently, much more data about patients’ medical histories exists in isolated healthcare IT systems than care providers can access when they need it.
The solution to this problem is to implement data integration across the industry. However, for a variety of reasons, this is a significantly more challenging task in healthcare than in other industries.
This guide explains what data integration is and the challenges it presents in healthcare.
Key Takeaways:
- The healthcare industry accumulates new data at a staggering rate.
- Care providers need real-time visibility into patient data to provide the best possible care.
- Data integration will enable higher standards of patient care, but not before the industry determines how to solve several critical challenges.
What Is Data Integration?
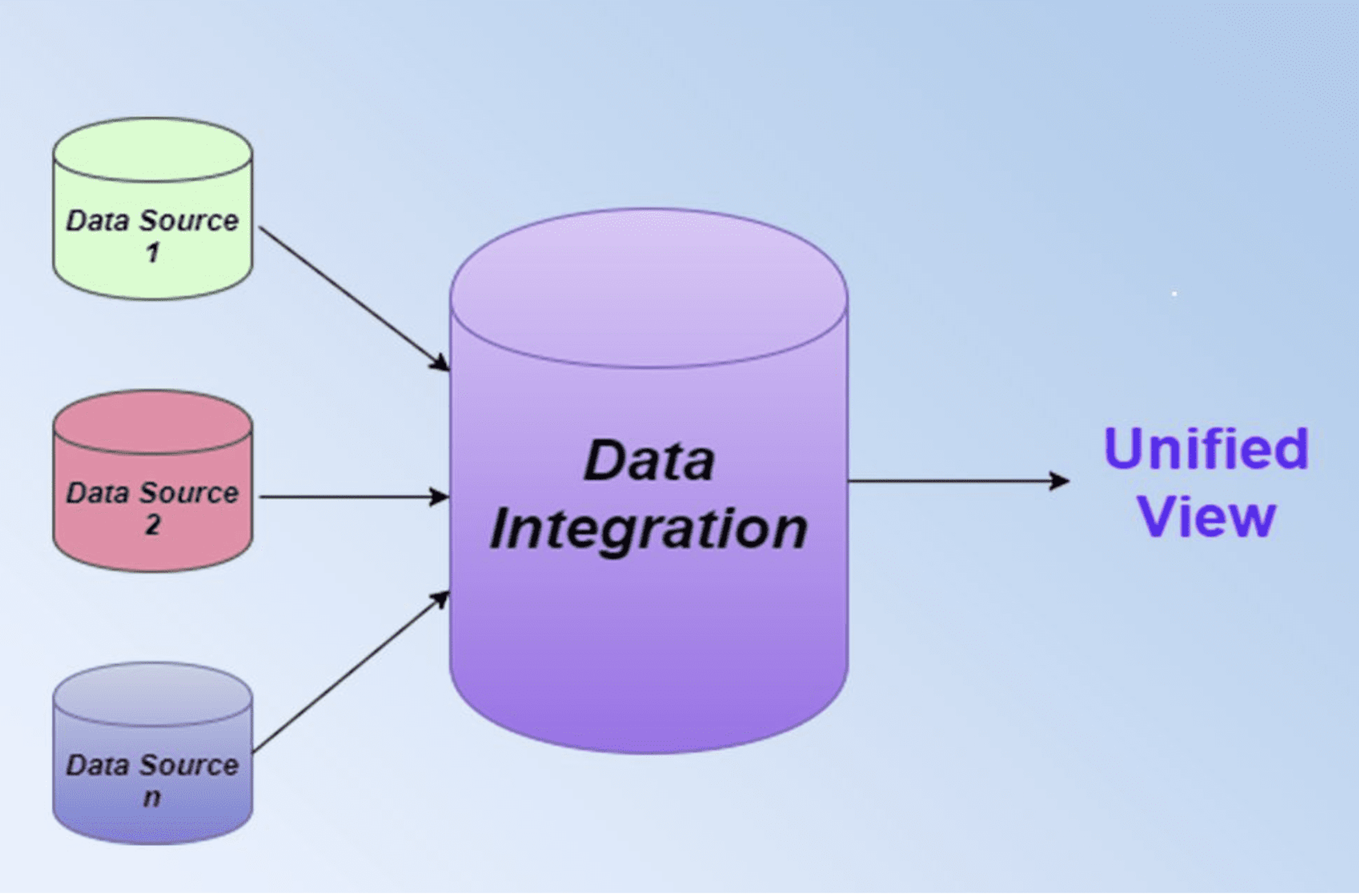
Image Source: Appsierra
Data integration refers to the process of combining data from various source systems to yield unified sets of information for both operational and analytical purposes. Data integration is a key component of the overall data management process. The purpose of data integration in an IT environment is to create consolidated data sets that are clean, consistently formatted, searchable, and suited to the data-use requirements of different end-users throughout an organization.
Data integration allows organizations to consolidate all the data they have about something – a user, an account, a total count for an action or process – onto a single screen. Integrated data feeds into applications that run day-to-day processes and passes into storage in data warehouses and lakes for future analytical and business intelligence (BI) uses.
Data integration involves a variety of techniques that attempt to reconcile discrepancies in how different systems store and format data about the same kinds of things. Depending on the systems involved, integration may happen at intervals – what data scientists call batches – or in continuous streams that update constantly as systems create new data.
Top 4 Healthcare Data Integration Challenges
Healthcare data integration faces similar challenges to commercial enterprises. Nevertheless, the industry’s unique regulatory requirements and the complexity of constituent organizations separate the data management needs of healthcare organizations from those of other businesses.
1. Lack of Standardized Formatting
The healthcare system includes a diverse range of organizations such as:
- Hospitals
- Clinics and private practices
- Medical imaging and radiology centers
- Dialysis centers
- Nursing homes
- Hospice care centers
- Birth centers
- Mental health facilities
- Physical therapy centers
These organizations store and format data in different ways. This makes integration a process that must incorporate potentially dozens of systems while consistently matching data to patients and correctly identifying duplicate records.
Healthcare data is also diverse even within individual organizations and includes:
- Text
- Images
- Charts and graphs
- Videos
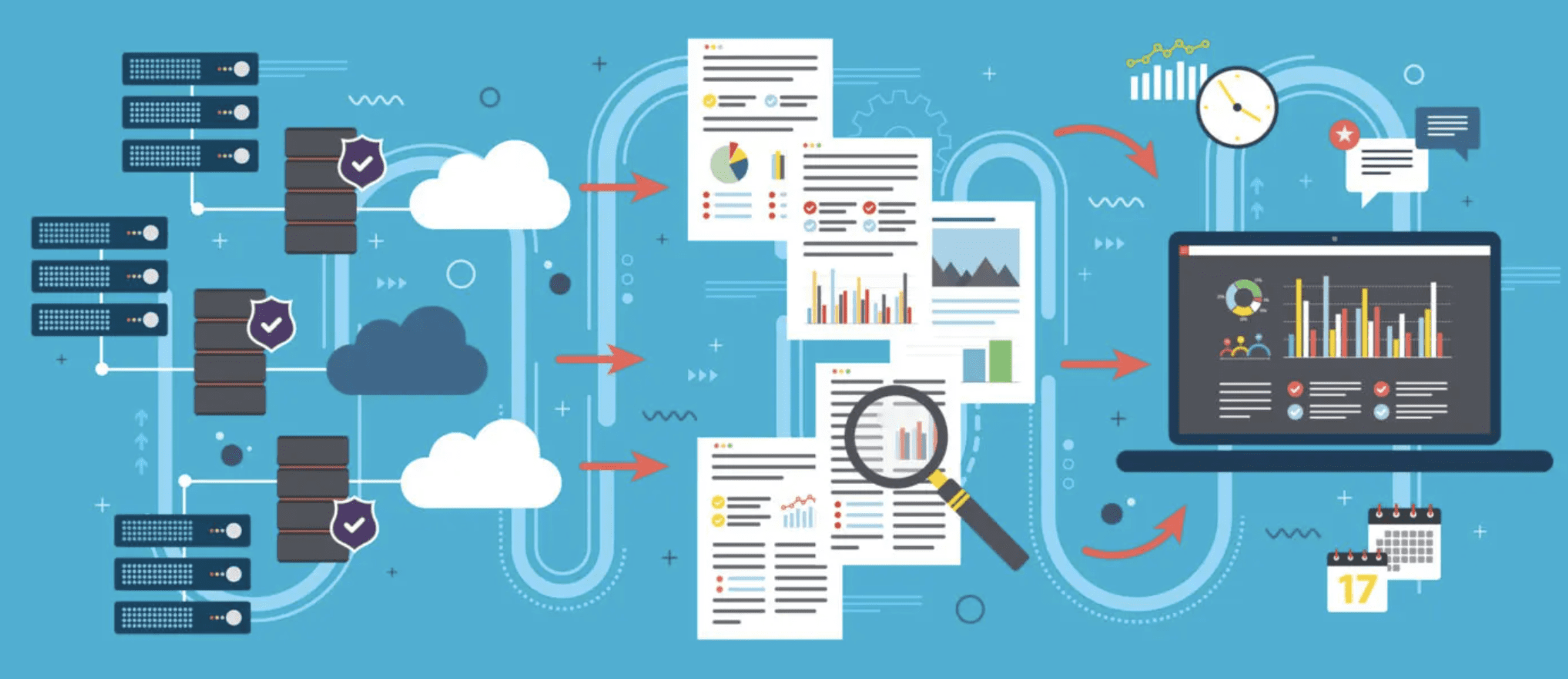
Image Source: Cioinsight
Moving data from unstructured sources such as images and video to ordered, searchable databases involves either costly and error-prone manual data entry or custom machine learning algorithms that can comb large data volumes without direct supervision.
2. Data Regulations and Privacy
In the U.S., two data privacy regulations dictate how healthcare organizations must store, share, and restrict access to patient data. These are the Health Insurance Portability and Accountability Act (HIPAA) and the Health Information Technology for Economic and Clinical Health Act (HITECH).
The HIPAA components that apply to patient data are:
- Security rule: Covers data integrity, confidentiality, and availability. Healthcare organizations must apply sufficiently sophisticated protections, such as encryption and multifactor authentication, to health records.
- Privacy rule: Gives patients control over how organizations use, retain, and dispose of their data.
HITECH aims to ensure patients have access to their electronic protected health information (ePHI). To comply with HITECH, organizations must guarantee that patients:
- Can access their ePHI.
- Approve access for third parties.
- Apply access exclusions for treatment, payment, and healthcare operations.
For healthcare organizations to integrate data in compliance with both regulations simultaneously requires balancing necessary partitions that ensure privacy and protection with sufficient communications between systems to enable data availability for patients.
3. Insufficient Human Resources
A lack of sufficiently experienced and credentialed personnel also presents a roadblock to healthcare data integration. In traditional commercial enterprises, dedicated IT staff likely require little direct supervision to make sense of different kinds of data and design appropriate integration pathways.
However, interpreting healthcare data often requires industry-specific training and credentials. As a result, data integration for healthcare requires an abnormally high number of specialized staff. As healthcare data is also exceedingly vast, keeping the necessary human resources on the job quickly becomes cost-prohibitive for many organizations.
4. Insufficient IT and Data Processing Resources
Many healthcare organizations continue to rely on legacy on-premises systems. Several reasons such as cost and compliance obligations contribute to the industry’s lag in cloud adoption.
Cloud-based computing and storage resources are crucial to processing large data volumes and creating the kind of interoperability that the healthcare industry is currently struggling to deliver. Many cloud data management platforms offer tools to protect and integrate data simultaneously and offload labor-intensive tasks to artificial intelligence (AI).
The challenge now will be convincing the necessary decision-makers to commit to the daunting upfront costs of adoption.
Real-Time Healthcare Data Integration with Coperor by Gaine
Coperor is a master data management platform designed for the healthcare industry. Using a profile-based operational data store (ODS), Coperor enables seamless integration of master data and affiliated data in real time.
To learn more about the Coperor Platform by Gaine, watch this demo of the platform in action.