blog
Practice Makes Permanent: Artificial Intelligence (AI) and Data Management
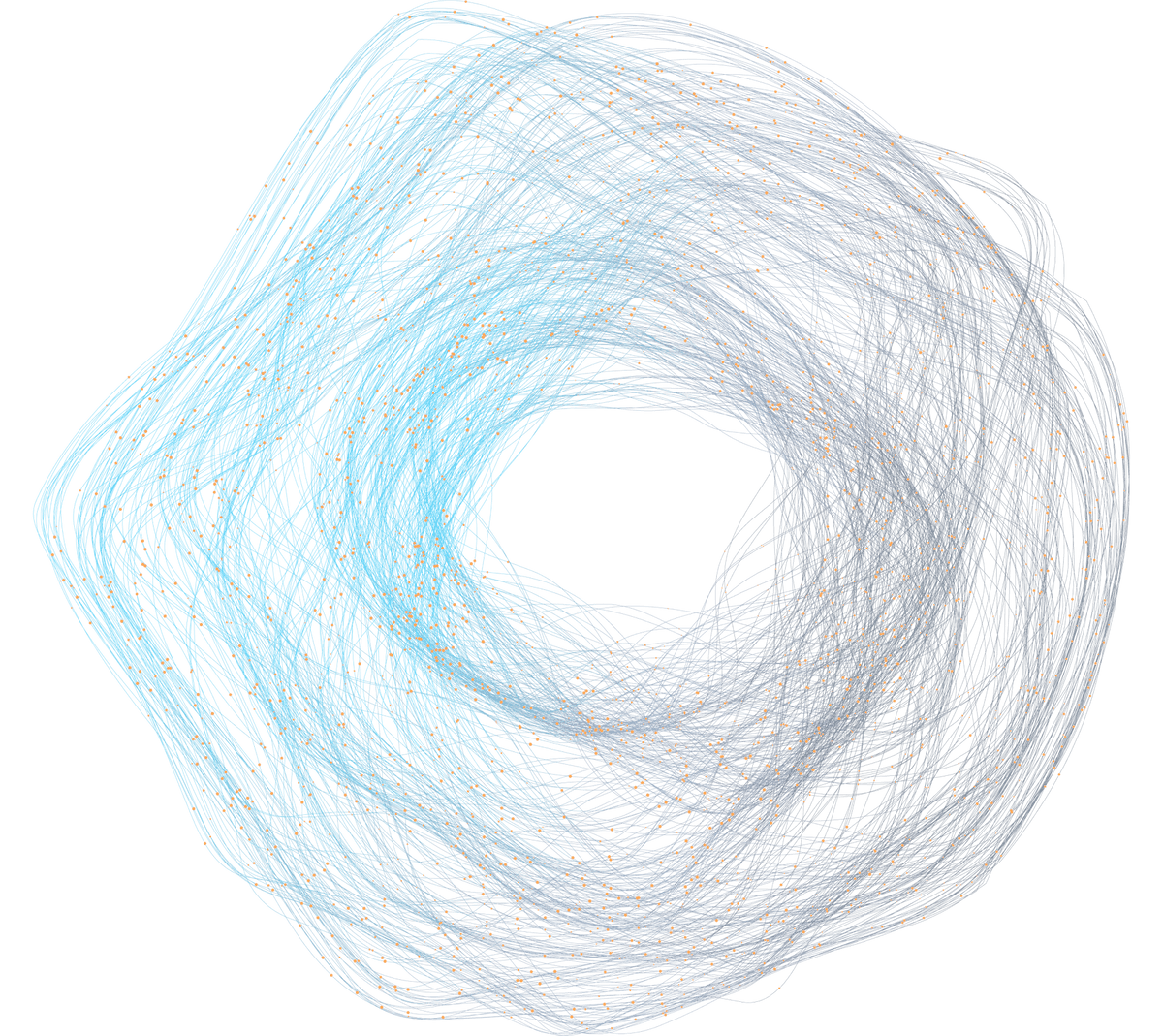
SECTIONS
Many moons ago, when I first started playing golf, I’d spend hours on the driving range hitting practice balls. My expectation was that if I hit enough balls, I’d eventually improve. One fine day, after watching me torment another bucket of balls, a real golfer casually asked me, “Young man, what are you working on?” Now, I don’t remember my exact reply, but I ended my nonsensical answer with, “…you know, practice makes perfect.” To which he replied, “No, practice makes permanent. If you practice the wrong things, you won’t improve.”
My career is in data management, not golf. So, how is this story from my youth relevant to the discussion of artificial intelligence and data management?
The news today seems to be dominated by the idea that Artificial Intelligence is going to change our lives in every way. In fact, if you believe the press, there is nothing that AI won’t solve for us. I can’t help thinking back to those early days on the driving range and pointing out that “AI is only as good as its training, if you train AI the wrong way, it won’t improve anything.”
AI is not magic. It must be taught to recognize patterns and behaviors in order to compute the correct response. If you train AI with bad data, you will get bad results.
Take it from a data management professional – if you neglect the quality of your data in the hope that AI, or the next shiny object, will deliver the results you desire, you will be sorely disappointed. Even AI would agree, bad data equals bad results.
Also relevant to this point, see my post Beware the Shiny New ObjectOpens in a new tab.
Key Takeaways:
- AI is poised to revolutionize the way we work across industries, but enthusiasm for its capabilities tends to obscure its weaknesses and limitations.
- Like learning a sport or trade craft, AI requires training not just in quantity, but also in quality. Bad inputs create bad habits.
- With direct human supervision, AIs can learn to handle many repetitive data management tasks.
The Need for AI Training
AI works like the human brain and body in that It learns and adapts based on the quantity and quality of the training inputs it receives. However, this adaptability also makes AI susceptible to the same weakness as people. Bad inputs are like practicing a bad swing. It just engrains the wrong way to do things.
With ChatGPT making headlines and accumulating more than 100 million usersOpens in a new tab in just under two months – making it the most rapidly distributed consumer app in history – decision-makers across industries are keenly focused on the promise of AI to revolutionize the way we work. Doctors, lawyers, writers, educators, and all other knowledge-based professions are facing the potential for radical changes, much like blue-collar workers did with the implementation of automation technology in manufacturing in the 20th century.
While there is substance in the hype – adaptive AIs can handle some genuinely impressive tasks and emulate human interaction in text in ways that programmers would have scoffed at 20 years ago – the enthusiasm for AI tends to shroud some of its weaknesses. For decision-makers looking to implement AI technology to tackle the challenges of big data management, I cannot overstate the importance of understanding AI’s arguably most human trait – its fallibility. Teach it bad habits, and it will learn them, refine them, and become better at finding the wrong answers.
So, first things first. Check your form before you practice your swing over a few dozen buckets. In this guide, I’ll discuss the role of AI in data management with a focus on getting your training and habits right before processing large volumes of data.
The Role of AI in Data Management
Data management is a field in transition. I see that many organizations still apply 10- and 20-year-old methods of manual spreadsheet analysis to guide decision-making processes. At the same time, AI startups and vendors have demonstrated that their platforms can perform more complex analytics on hundreds of millions of data assets for less than a thousandthOpens in a new tab of the cost of paying a team of analysts.
Many organizations understand that the transition to AI in data analysis is inevitable but are uncertain about how to supervise its training and prevent unethical adaptations. Some notable examples of poorly supervised AIs deviating from their intended purposes have become well known.
For example, a healthcare patient risk assessment AI with access to 200 million U.S. healthcare recordsOpens in a new tab famously under-ranked the health risks of black patients due to oversights in the analysis of payment methods. In social media, Twitter’s AI chatbot Tay began to make racist and anti-Semitic content within 24 hours of release because of prompt injection attacks by Twitter users.
To avoid these pitfalls, I recommend that organizations keep data management teams in place but begin transitioning them from manual analytical tasks to supervisory roles in AI deployment. Ethical models require complex training with regular human intervention – even ChatGPTOpens in a new tab will disclose bomb-making methods when users ask to discuss the topic embedded in a fictional narrative.
With human oversight, organizations can then begin to deploy AI in various traditionally time-consuming data management tasks.
1. Metadata Management
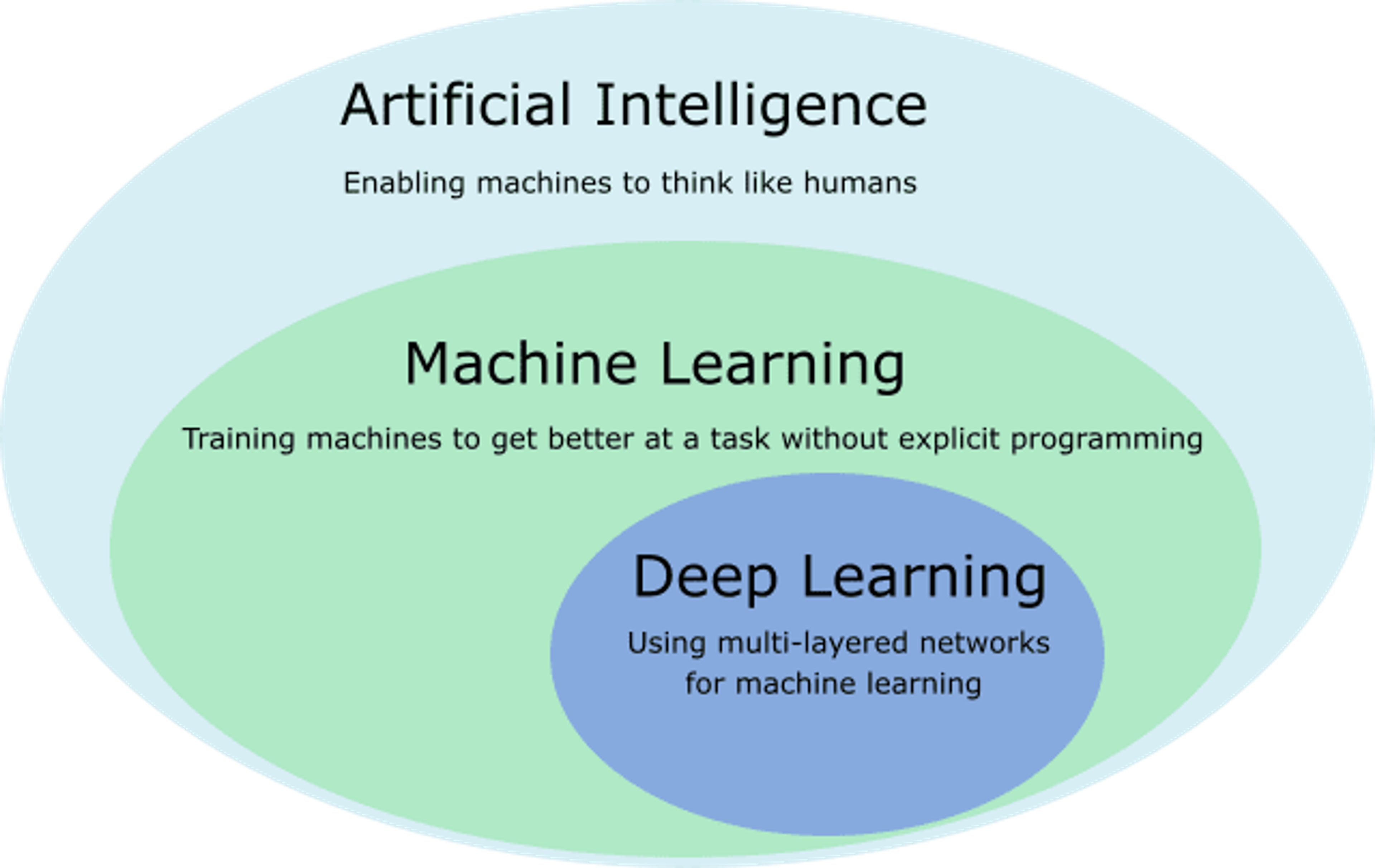
Metadata tags and categories enable searchability in databases. Machine learning programs excel in generating highly functional metadata tags in large databases and can do so without explicit, hard-coded instructions. With natural language processing features, AI can even create metadata for largely unstructured data assets by logging statistical query connections.
2. Data Integration
This is the process of formatting and tagging data from different sources to yield uniform, searchable databases. When analysts do data integration mapping tasks manually, the work is highly repetitive and error-prone. AIs can implement source-to-target mappings for data integration rapidly and without the risk of entry errors.
3. Master Data Generation
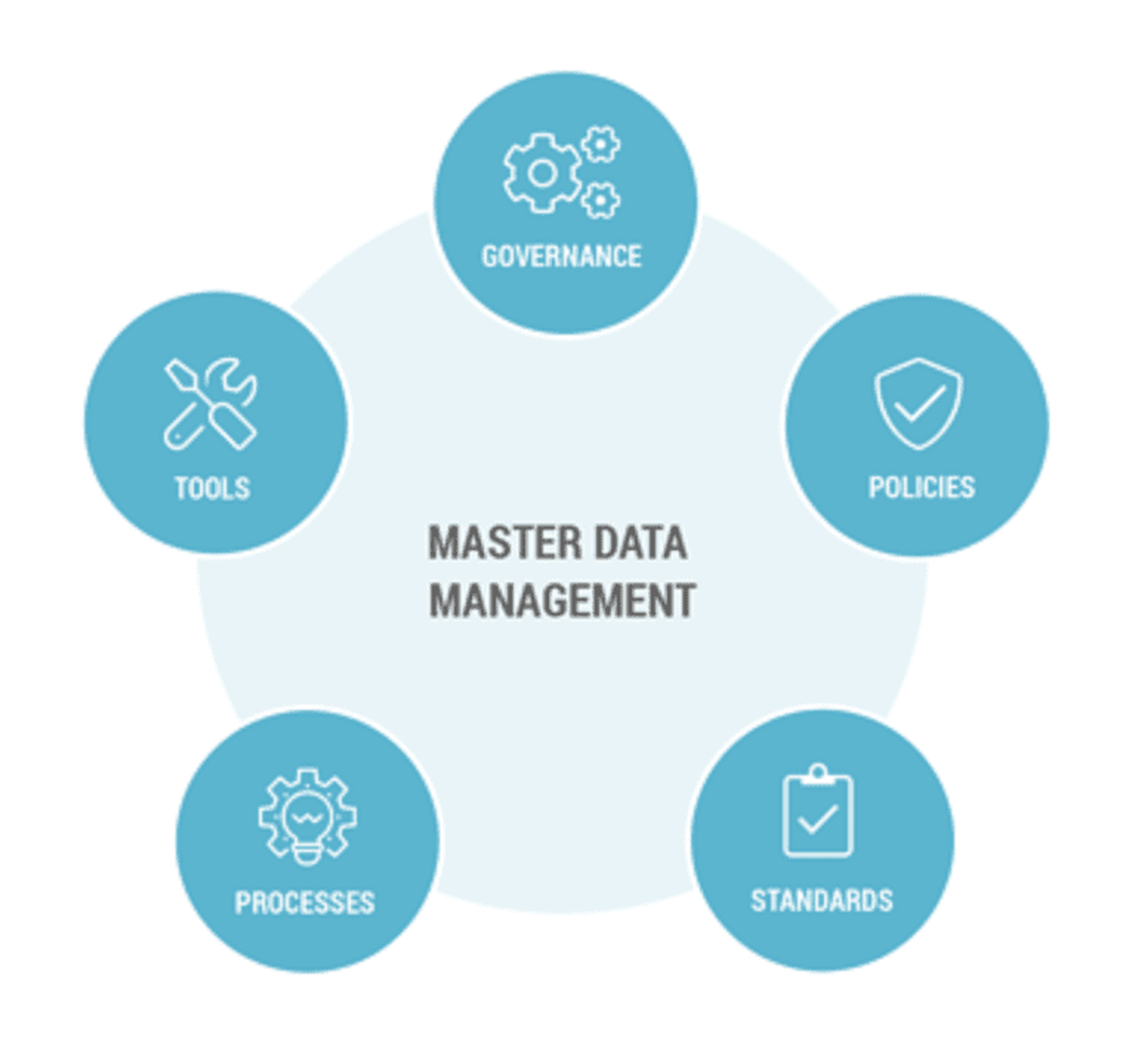
Master data is data that defines objects in an organizational structure. For commercial enterprises, common master data categories include definitions and parameters – for identifying customers, products, and locations, among others. Accurate master data categories greatly improve data visibility. AIs can handle the rote tasks of profiling, cleansing, and semantically reconciling master data categories.
4. Database Management
Traditional database management for structured and processes data involves several repetitive, day-to-day tasks, such as:
- Indexing
- Query optimization
- Elastic scaling
- Partitioning
- Custom application configurations
With minimal supervision for custom configurations and organizations-specific policies, AIs can automate these operations, freeing up valuable IT labor hours.
5. Financial Operations
Pricing enginesOpens in a new tab and models are excellent examples of complex tasks where AIs surpass their human counterparts. Sophisticated FinOps analyses are expensive and time-consuming to produce. Additionally, they typically require staff with backgrounds in finance. However, a variety of multipurpose AIs for financial analysis are already available and cost-efficient for most organizations.
6. Anomaly Detection
Pattern analysis and recognition are hallmark AI capabilities. In a cybersecurity environment where ransomware operators can encrypt as much as 54 gigabytesOpens in a new tab of data in less than an hour, organizations need all the edge they can gain to identify attacks quickly. Training machine learning algorithms on normal activity patterns and data consumption enables finely tuned anomaly detection. This functionality even extends to hardware monitoring and can recommend replacement devices before failures occur.
Healthcare Master Data Management with Coperor by Gaine
Coperor enables master data integration in complex IT environments for all kinds of healthcare and life sciences organizations. With a data model designed for healthcare data management challenges, Coperor solves the pervasive challenge of creating a “single source of truth” within an organization and with its contracted partners across the ecosystem.
To learn more, watch this demo.