blog
Operational Data Store Vs. Data Warehouse: What Works Better?
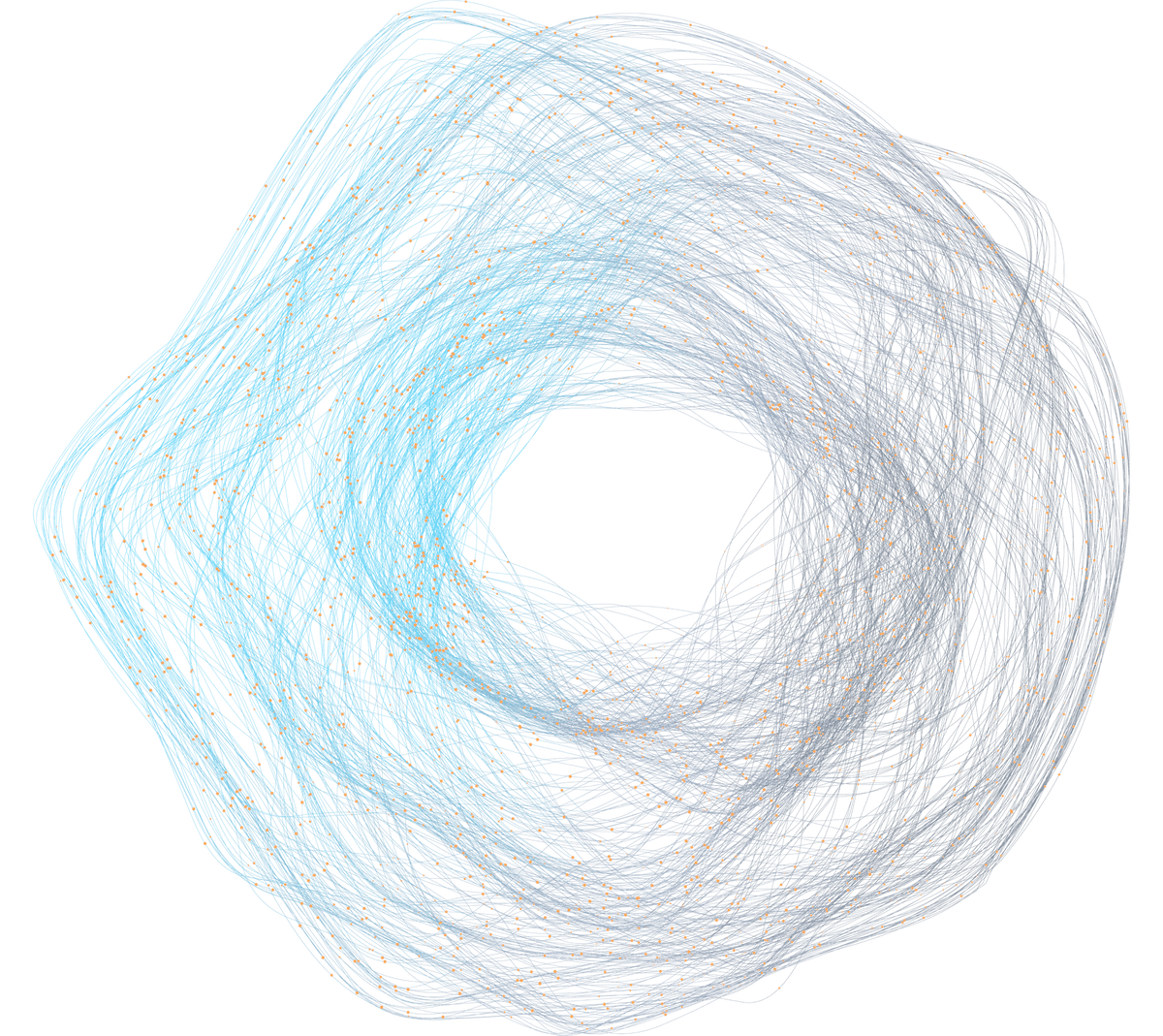
SECTIONS
Operational data store vs. data warehouse—which is the better option? It’s an important question in the healthcare and life sciences sectors, where effective data management is a cornerstone of quality care, research, and innovation. From drug discovery and clinical trials to maintaining regulatory compliance and gaining market insights, these sectors rely heavily on data to drive advancements and deliver outcomes.
But the vast volumes of complex and diverse healthcare data generated every day necessitate a robust framework for data management to ensure data integrity, privacy, security, and compliance. The decision between utilizing an operational data store (ODS) and a data warehouse is thus a critical one.
To navigate this decision, organizations must consider their specific needs related to real-time data access, reporting requirements, and long-term data analysis. The right choice depends on the nature of a company’s data, its intended use, and scalability requirements, among other factors.
This guide will prove a thorough comparison between an operational data store vs. data warehouse, helping healthcare and life sciences organizations make an informed decision that aligns with their data management strategies, existing infrastructure, and overarching goals.
Key Takeaways:
- Both Operational data stores and data warehouses are essential, serving complementary roles in healthcare and life sciences data management.
- An ODS offers real-time data integration for operational support, while a data warehouse provides historical data analysis for strategic decision-making.
- Utilizing both systems allows organizations to meet both immediate operational needs and long-term analytical requirements effectively.
- A tiered data management approach, where data flows from an ODS to a data warehouse, maximizes data utility and supports comprehensive analysis.
- Integrating ODS and data warehouse strategies ensures operational efficiency and enhances strategic depth in data analysis for healthcare and life sciences organizations.
What Is an Operational Data Store?
An operational data store is a centralized database that aggregates data from multiple sources to provide a current snapshot of business operations. It is designed for routine activities such as reporting, analysis, and operational support, facilitating timely decision-making and operational reporting.
The ODS is dynamic, frequently updated to reflect the latest state of data across the organization, making it a vital component in the data management ecosystem, especially in fast-paced environments like healthcare and life sciences.
Key Features of an ODS:
- Real-time or near-real-time data integration: Ensures that the data is current, reflecting the latest operational activities.
- Data consolidation: Aggregates data from various sources into a unified format, making it easier to access and analyze.
- Support for operational reporting: Enables quick, ad-hoc reports and queries to support day-to-day business operations.
- High data quality and consistency: Provides a clean, consolidated view of data, improving the reliability of operational processes and decisions.
Typical Use Cases in Healthcare:
- Patient care and management: An ODS can offer healthcare providers a comprehensive, up-to-date view of patient records, treatment histories, and care plans.
- Operational reporting: Hospitals and clinics use ODS for real-time reporting on patient flow, resource utilization, and operational efficiency.
- Compliance and monitoring: Ensures that healthcare organizations can quickly access and report data to comply with regulatory requirements.
Advantages:
- Improved decision-making: By providing real-time or near-real-time data, an ODS enables healthcare professionals to make informed decisions quickly.
- Enhanced operational efficiency: Streamlines operations by consolidating data from various sources, reducing redundancy, and improving data quality.
- Flexibility: Can be updated or modified more easily than a data warehouse to adapt to changing operational requirements.
Potential Drawbacks:
- Complexity in integration: Aggregating data from multiple sources can be complex and challenging, requiring robust integration tools and expertise.
- Maintenance: Requires ongoing maintenance to ensure data remains current and accurate, which can be resource-intensive.
- Scalability issues: While designed for operational efficiency, an ODS might struggle to scale with massive volumes of data or extremely high query loads, potentially affecting performance.
In summary, an Operational Data Store serves as a critical component in healthcare data management, offering timely insights into operational data to support decision-making and efficiency. However, organizations must carefully consider the integration, maintenance, and scalability challenges associated with successful implementation.
What Is a Data Warehouse?
A data warehouse is a centralized repository designed to store integrated data from multiple sources over a long period. It supports the consolidation, analysis, and reporting of historical data, facilitating strategic decision-making.
Unlike an operational data store that focuses on current operations, a data warehouse is optimized for batch processing and complex queries across large datasets, making it essential for data-driven strategies in various sectors, including healthcare and life sciences.
Key Characteristics of a Data Warehouse:
- Historical data storage: It archives data over extended periods, enabling trend analysis and historical insight generation.
- Subject-oriented: Organized around major subjects, such as patients, treatments, or outcomes, rather than specific operations.
- Integrated: Consolidates data from various sources into a coherent whole, ensuring consistency and accuracy.
- Non-volatile: Once entered into the warehouse, data is not updated or deleted, preserving its state for accurate historical analysis.
Common Use Cases in Healthcare and Life Sciences:
- Research and development: Facilitates the analysis of extensive datasets to identify trends, patterns, and correlations that can inform new drug development or medical treatments.
- Regulatory compliance reporting: Stores comprehensive historical data necessary for regulatory reporting and audits.
- Patient outcomes analysis: Enables the longitudinal study of patient data to assess treatment effectiveness and improve patient care strategies.
Advantages:
- Informed decision-making: Provides a solid foundation for strategic planning and decision-making by offering access to historical data and comprehensive analytics.
- Enhanced data quality and consistency: Through data integration and cleansing processes, it ensures high-quality, consistent data for analysis.
- Scalability: Designed to handle large volumes of data, making it suitable for organizations that need to store and analyze data over long periods.
Possible Limitations:
- Complexity and cost: Setting up and maintaining a data warehouse can be complex and costly, requiring significant investment in technology and expertise.
- Inflexibility: The structured nature of a data warehouse can make it difficult to adapt to new data sources or analytical needs quickly.
- Delayed data access: Due to its focus on batch processing, there may be delays in data availability, making it less suitable for real-time decision-making.
In essence, a data warehouse plays a pivotal role in the life sciences sector by enabling comprehensive analysis of historical data to drive research, compliance, and patient care improvements. While offering significant benefits in terms of data quality and analytical capabilities, organizations must navigate its complexities, costs, and potential inflexibilities to fully leverage its potential.
How Operational Data Stores and Data Warehouses Work Together
When it comes to data management, particularly within the healthcare and life sciences sectors, it’s not about choosing between an operational data store vs. data warehouse but rather utilizing both to harness their complementary strengths. This dual approach enables organizations to optimize their data management strategies, ensuring both operational efficiency and strategic depth in data analysis.
An ODS provides the data access and integration crucial for day-to-day decision-making, while a data warehouse stores high-volume historical data, allowing for complex queries, trend analysis, and strategic planning over time.

Image Source
In other words: You can see the forest and the trees, zooming in and out as needed to leverage your data for the exact situation at hand.
In a complementary setup, data flows from each disparate source into the ODS for immediate use. Here, the data can be cleaned, transformed, and loaded into the data warehouse for long-term storage and analysis. This tiered approach ensures that data serves both immediate operational needs and strategic analysis, maximizing its utility.
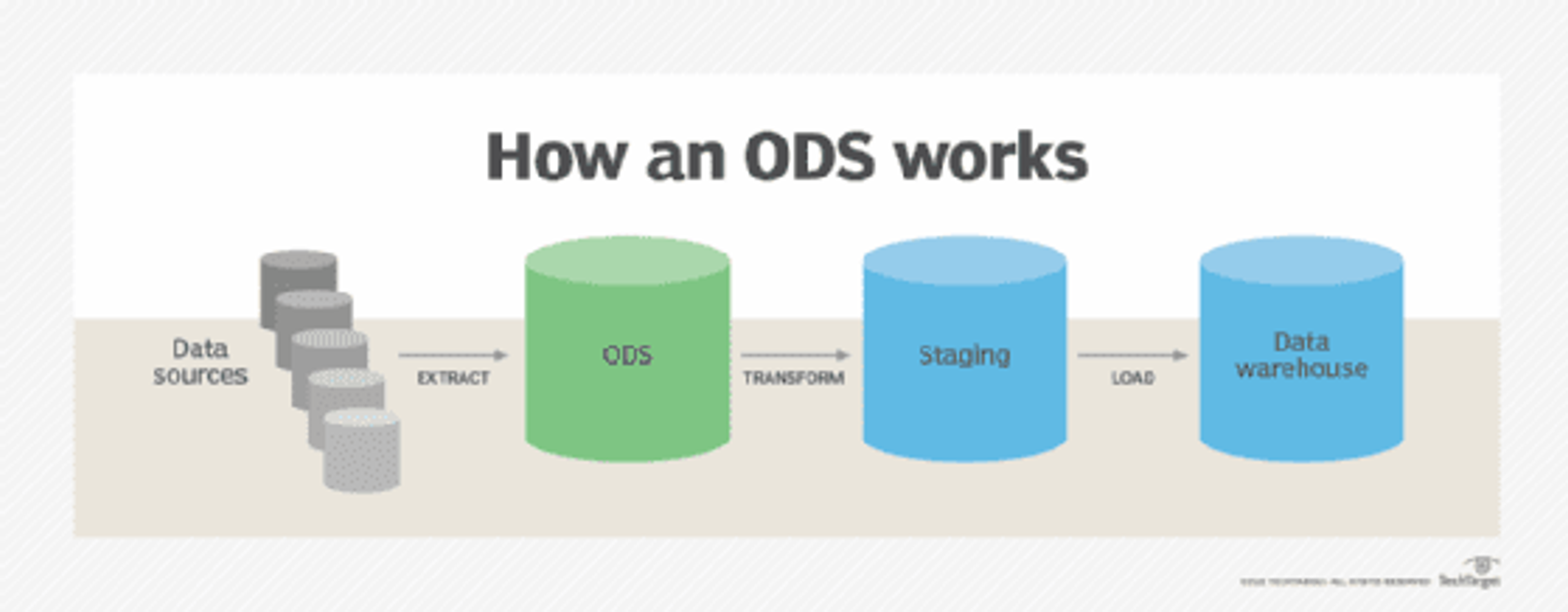
Image Source
By leveraging both an ODS and a data warehouse, organizations can make informed decisions at every level. The ODS can manage the load of real-time queries without impacting the performance of the data warehouse, which can be optimized for heavy, complex analytical queries.
Modern technology tools help to facilitate the seamless integration of an ODS with a data warehouse, especially now that cloud-based platforms offer scalable and flexible environments that can support both ODS and data warehouse functionalities.
These tools not only streamline the data transformation and loading processes but also offer advanced capabilities for data governance, quality control, and real-time analytics.
Putting It All Together
Operational data stores and data warehouses aren’t mutually exclusive. Rather, they’re complementary tools in the data management toolkit. Together, they provide a comprehensive solution that supports both the immediate operational needs and the strategic analytical requirements of healthcare and life sciences organizations.
By integrating both systems into your data management strategy, your organization can ensure that it is always prepared to meet immediate data needs, while handling its data effectively for long-term storage, compliance, and data analytics ROI.
Gaine provides ecosystem-wide data management solutions. Our proven industry data model has expanded and matured over many years and multiple projects to deliver reliable results across dozens of complex use cases in healthcare and life sciences.
Learn more here about our innovative solutions stack.