blog
Data Quality: Beyond Standardizing Values and Improving Fill Rates
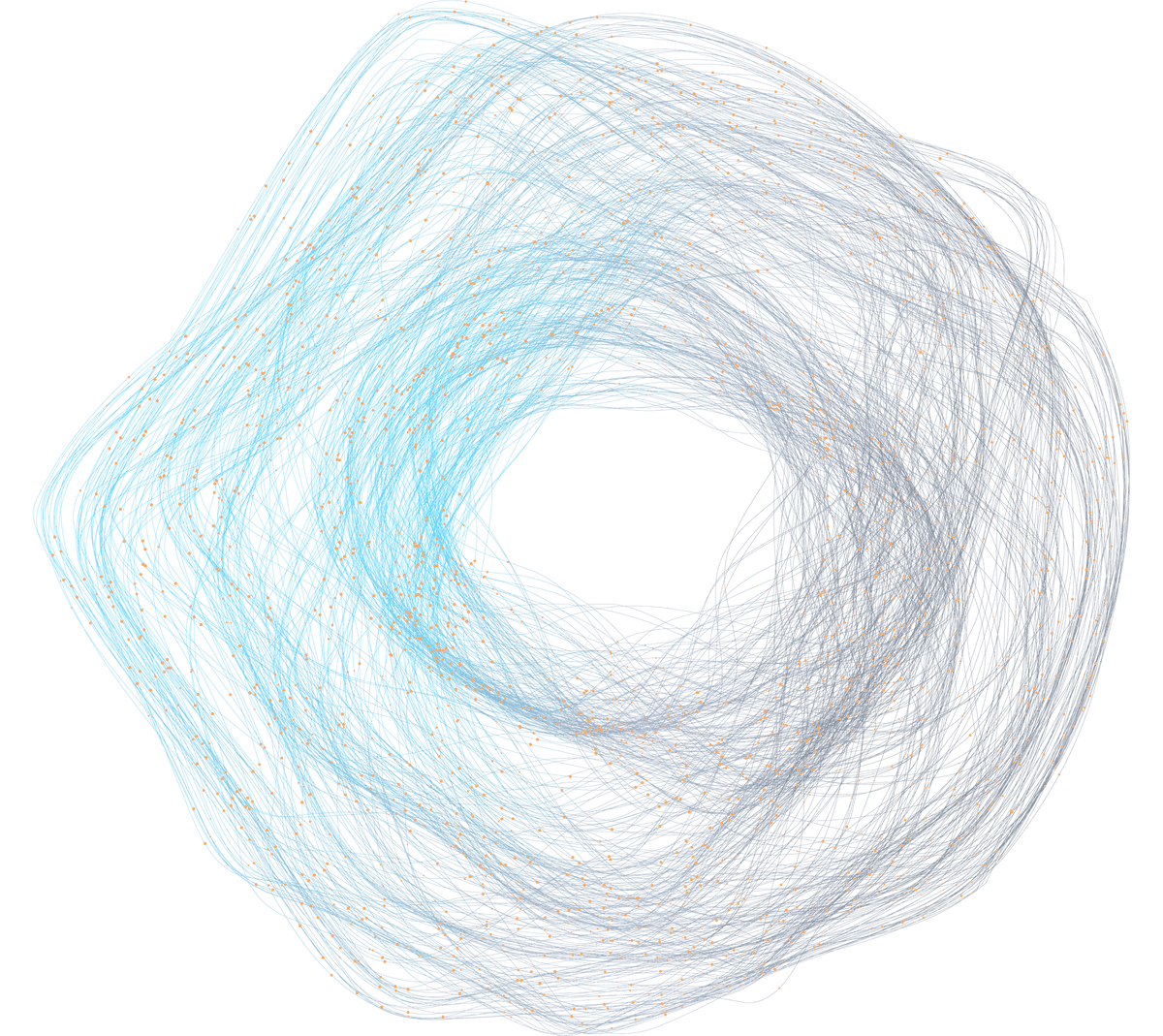
SECTIONS
The global datasphere – the total quantity of digital data generated by human activities – has been rapidly expanding, doubling in size every two years for the past decade. By the end of 2025, it will reach a total volume of 51 zettabytesOpens in a new tab – or 51 trillion gigabytes. As organizations strive to develop new capabilities for managing ballooning volumes of data and extracting value from them, many are also learning a hard lesson in the cost of poor data quality.
Recent studies have found that poor data quality costs organizations an average of $12.9 millionOpens in a new tab annually, equaling approximately 20% of their total revenue. To recoup these losses and reap the full value of data, organizations must first understand how to measure and improve data quality.
In this guide, you’ll learn what data quality is and what practices you can implement to enhance it in your organization.
Key Takeaways:
- The global datasphere is growing rapidly, doubling in size every two years.
- This newly captured data has a high potential value that most organizations fail to capture.
- Implementing a six-point data quality plan can help your organization raise data quality levels.
What Is Data Quality?
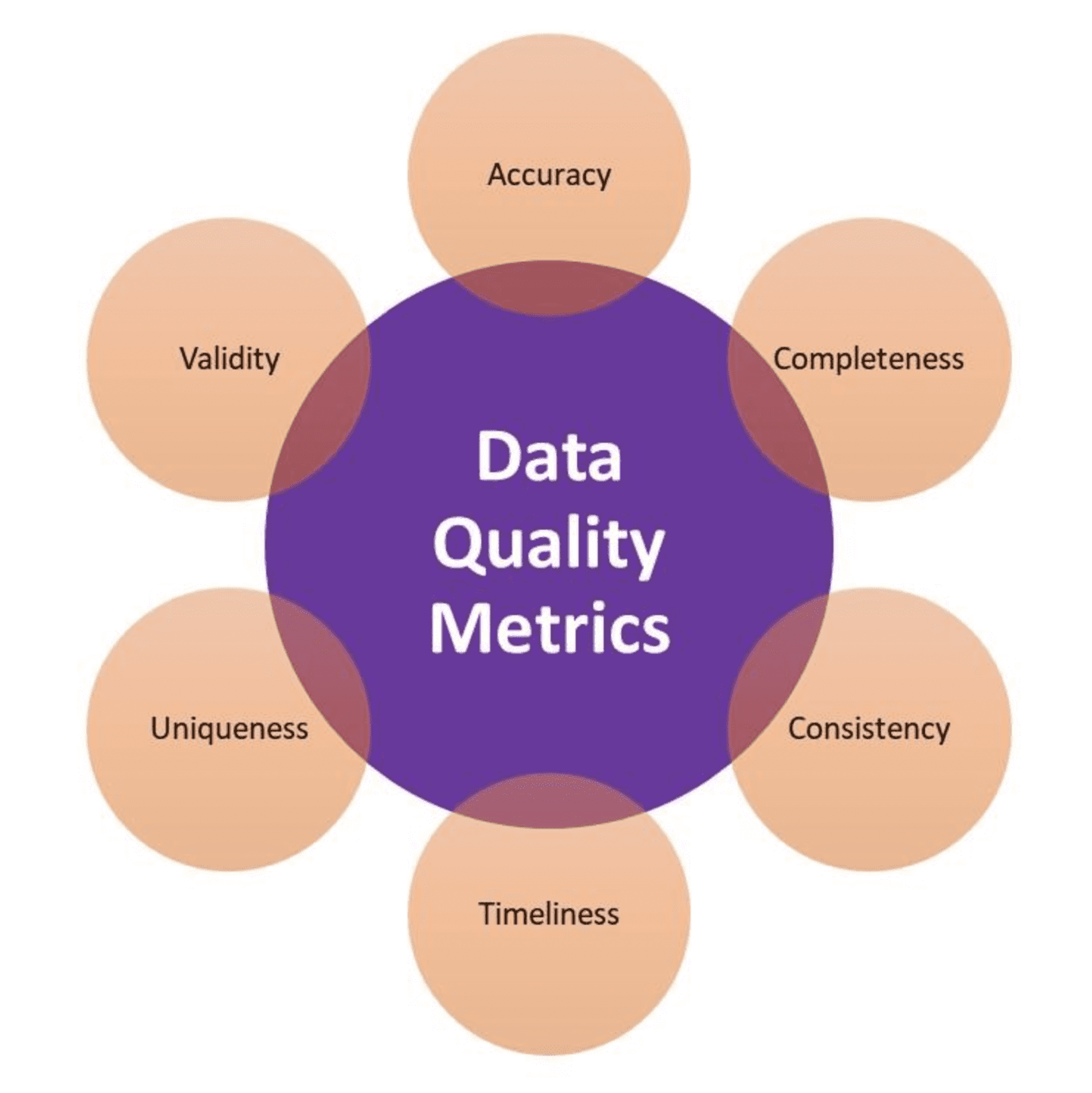
Image Source: FirstEigen
Data quality refers to factors affecting the condition and accessibility of data in an organization. While accuracy is a critical component of data quality, several other important factors contribute to the end state of data for users. To generate value and guide business decisions, data must be accurate, comprehensive in scope, and easily accessible to those who need it.
To improve data quality, many organizations implement data management systems that standardize entry values and improve entry completion rates. These measures tend to improve overall data quality, but they are not sufficient on their own to consistently yield high-quality end-user data. Achieving that goal requires a more holistic approach.
While there are many lists of data quality characteristics in use in different industries, six characteristics have increasingly become standard in organizations trying to improve data quality. These are:
- Accuracy
- Completeness
- Relevance
- Consistency
- Accessibility
- Timeliness
Data management systems that target these objectives help organizations extract optimal value from the data they capture. With high values in each of these categories, organizations can expect reliable data insights that guide decision-makers to better bottom-line performance.
6 Characteristics of Data Quality
These six characteristics are widely recognized as indicators of organizational data quality.
1. Accuracy
In data management, data accuracy is the rate at which recorded values are true. Errors or inaccuracies can enter data management systems via several pathways, such as through manual data entry or due to formatting issues.
For example, differences in date formatting, such as the order of month, day, and year in the U.S., versus day, month, and year in most countries in Europe and Asia. In systems where values migrate automatically, these kinds of formatting discrepancies can cause widespread downstream data inaccuracies.
2. Completeness
Highly accurate data doesn’t generate value if it fails to include important value fields. For data management purposes, completeness is equally as important as accuracy. Gaps in data fields and data visualizationOpens in a new tab may render the entire category unusable.
For example, form entry fields for names that don’t match first and last names with other unique identifiers result in entries that don’t resolve to a single identity. To avoid such gaps in completeness, organizations must use data entry systems that require specific field validations to create entries and ensure all required data is captured.
3. Relevance
Like customer relationship management (CRM) software and enterprise resource planning (ERP) platforms, today’s IT systems have advanced capabilities for capturing all kinds of operational and transactional data. While it may be tempting for data managers to think of more as better, organizations must be mindful of the data they collect and evaluate its relevance and value.
Capturing excess data for fields with no operational relevance consumes valuable labor resources. Generating high-quality data requires mapping captured fields to downstream use cases.
4. Consistency
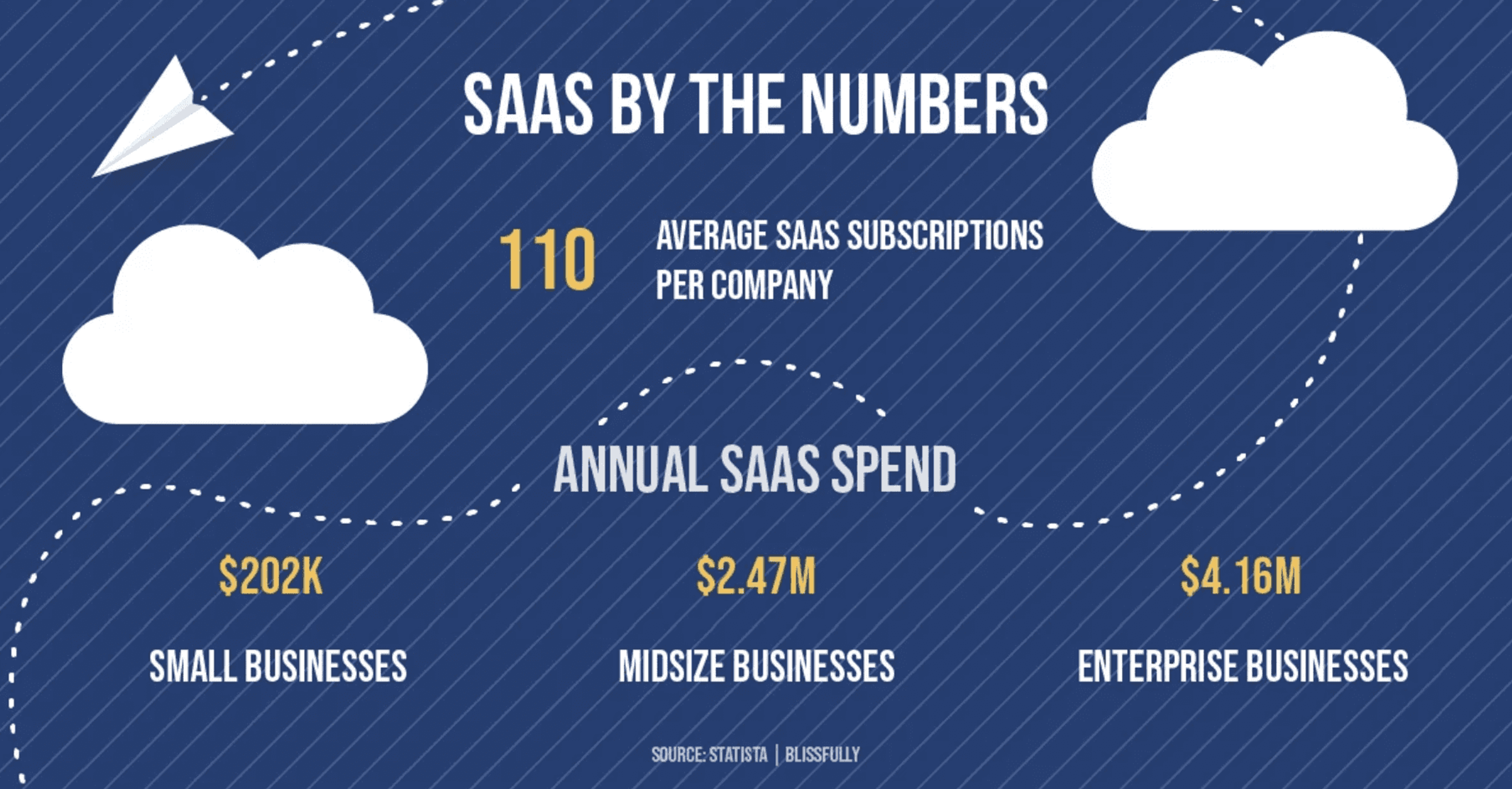
Image Source: Stampli
The number of software-as-a-service (SaaS) applications that companies use rises year over year, with enterprise-level organizations using an average of 110 SaaS platformsOpens in a new tab in 2022. This platform-heavy environment tends to create multiple potential sources for data inconsistencies.
If data enters your system at multiple manual entry points, redundant fields will likely end up with inconsistent entries. To reduce the chances of inconsistent data entries entering your systems, organizations can implement strict form validation fields integrated with master data management systems.
5. Accessibility
End-user data exists at many points. Users may pull it from a variety of CRM- and ERP-connected points. While many systems have automated data migrations at regular schedules, IT administrators should ensure these systems are configured properly and communicating effectively. (Data automation capabilities remain highly configurable.)
Most still require scheduled migrations. Administrators should also consider whether end users need structured or unstructured data. Reams of unstructured data may not resolve queries.
6. Timeliness
Data accuracy and completeness depend on timely delivery for the best value. If your system holds actionable insights for days to weeks before yielding workable outputs, its fidelity is a lost expense.
Outdated data can incur as much unnecessary cost as bad or incomplete data. To ensure data timeliness, IT teams must configure systems to report on a minimum 24-hour basis. High data quality requires narrow update frames across all systems to ensure the data remains relevant.
Master Data Management Capabilities Designed for Healthcare Organizations
Gaine’s industry-first master data management platform, Coperor tackles the unique data management challenges in the healthcare industry. With ecosystem-wide scalability and comprehensive field and object capabilities, Coperor enables systemic data integration for healthcare organizations.
Coperor allows for complex profile management and tight coupling of affiliated data, allowing users to differentiate endpoints with confidence. With an unrivaled healthcare-focused data model, Coperor creates solutions for the industry’s need for a single source of truth in data management.
To learn more about Coperor, contact Gaine today.