blog
Six Data Quality Checks Sanator Performs on Healthcare Provider Data
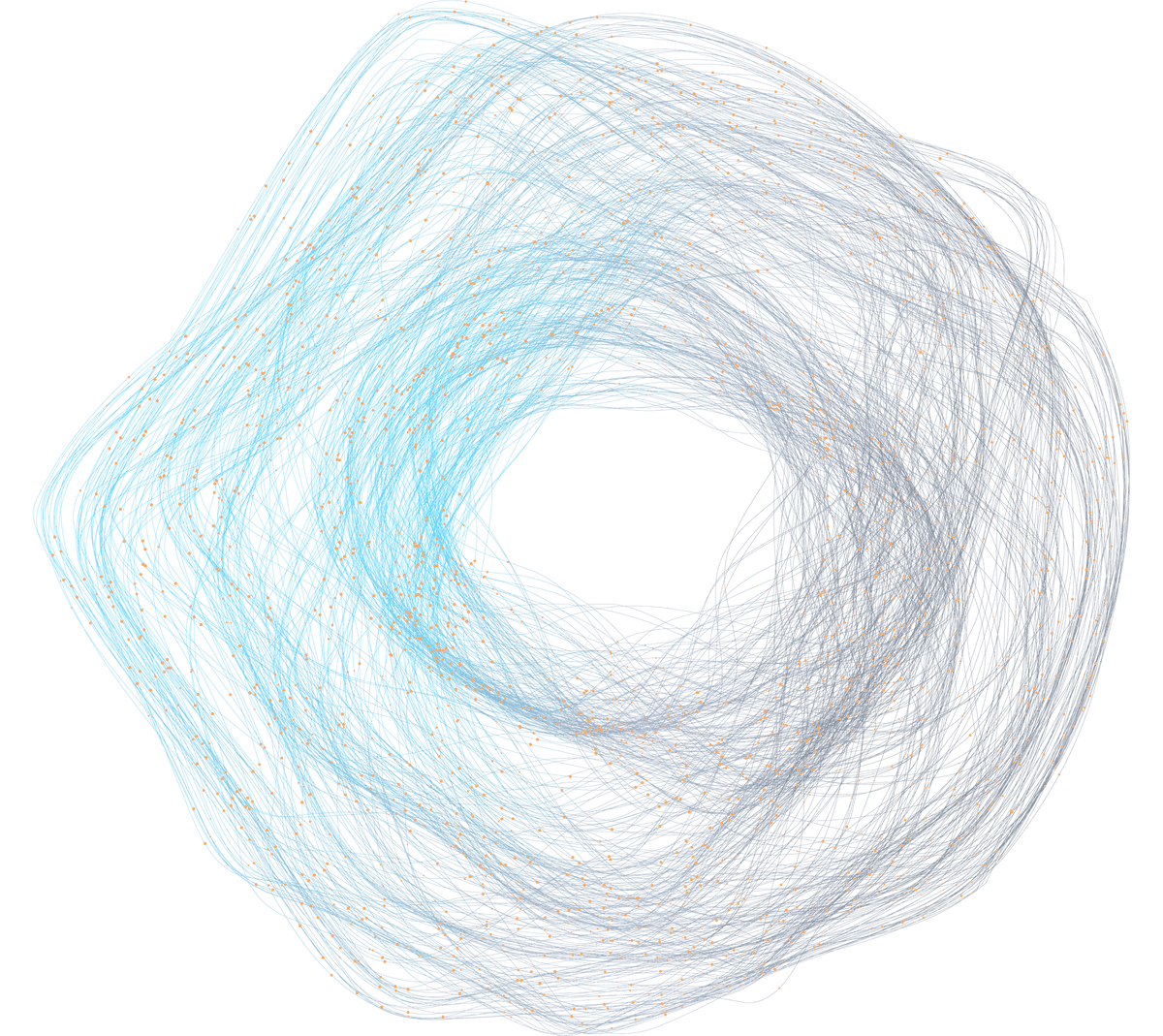
SECTIONS
The effects of poor data quality across all industries have the same thing in common: unreliable information could drive away customers or clients and result in a loss of revenue. However, in healthcare, data quality has the added and solemn function of ensuring proper patient medical care. Therefore, data quality checks in this industry are crucial.
A recent study showed that 55% of organizations lack trustOpens in a new tab in their data assets.
How can healthcare providers gain complete trust in their data? What checks should they be performing? We explore these questions.
Key takeaways:
- Four benefits of good data quality include superior patient care, employee trust in technology, increased efficiency, and data-driven decision-making.
- Completeness means the required values in a record shouldn’t have missing or partial data.
- Consistency means that fields within a record correlate with each other and that records across departments or facilities match.
- Also known as validity, conformity means that the data must adhere to a specified format, whether the country or the business dictates it.
- Uniqueness means there are no duplicate entries in the data.
What is Data Quality?
Data quality is a gauge of the condition of information based on six factors: accuracy, completeness, consistency, conformity, uniqueness, and timeliness. Assessing data quality levels can help organizations identify errors in the information that need resolution and determine whether the data in their electronic systems are suitable for its intended purpose.
Data quality checks in business have increased as data processing has become more connected with business operations, and organizations increasingly use data analytics to become data-drivenOpens in a new tab.
The Importance of Data Quality
Poor data quality causes complications for an organization, impacting everything from patient treatment to policy-level decision-making. Excellent data quality, on the other hand, has the following benefits:
- High-quality patient care – When source data is accurate in the electronic healthcare system, there’s no need for an individual to manually resolve discrepancies and inaccuracies, leading to delays and mistakes. Instead, the patient receives proper treatment and timely attention.
- Employee trust in technology – With good data quality, employees won’t distrust the electronic system and revert to manual reporting and data analysis.
- Increased employee efficiency – Since manual interventions to correct inaccurate data aren’t needed, automated work frees employees to handle more complex tasks.
Data-driven decision-making – Organizations rely on big data to help make business-level and policy-based decisions. Having high-quality data on hand aids good decision-making.
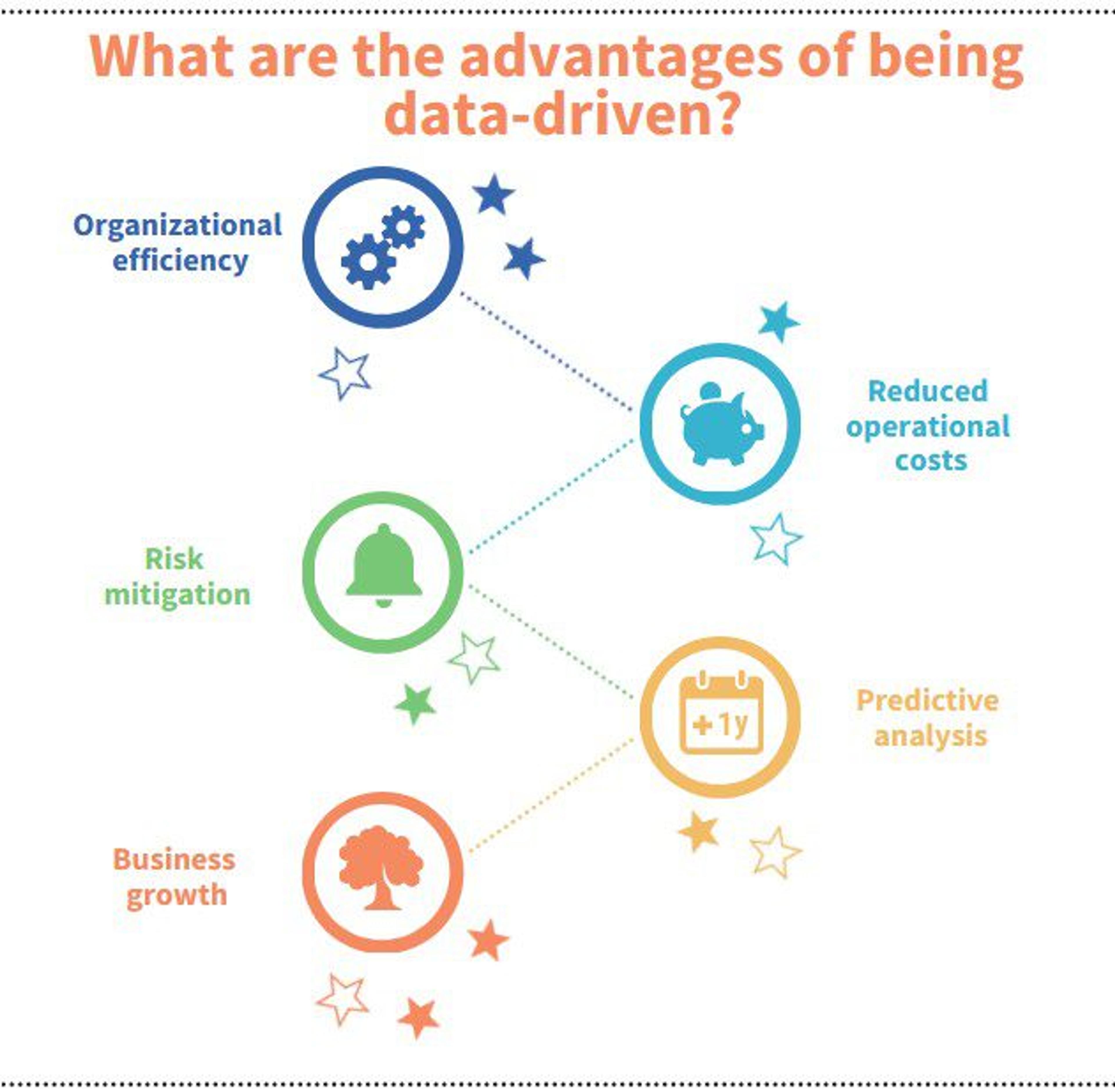
Image source: www.statsfind.com
The Fundamental Data Quality Checks
Now that we understand the value of good data quality, let’s examine the six quality checks that healthcare providers should continuously perform.
1. Accuracy
Check that the data is precise, ensuring every detail of a patient’s record is correct and acceptably presented. For example, is the patient’s name spelled correctly? Do you have the correct home address?
Verification when entering the data is vital for data accuracy. For instance, it’s common to have a patient verbally spell out their last name and confirm their date of birth. Verifying a patient’s insurance member ID may mean accessing the insurance company’s website, your management system, or making a phone call.
2. Completeness
The required values in a record shouldn’t have missing or partial data. For example, a patient’s first and last name are needed values, while the middle name may be optional. So, if a person’s name is Alexandra Smith, the field for the first name should say “Alexandra,” not “Alex“ nor “A.”.
Other critical values to check for missing in partial data are:
- age, height, and weight
- current and past diagnoses or surgeries
- current and past medications
- family history of diseases
- allergies
3. Consistency
Consistency means that fields within a record correlate with each other. For example, if the date of birth field says May 1, 1999, but the age field says 20 years old, the two pieces of data are inconsistent and need correction.
Consistency also means that records across departments or facilities match as well. For example, if the date of birth field reads May 1, 1999, in the medical records but reads October 1, 1999, in the accounting records, these too are inconsistent and need correction.
4. Conformity
Also known as validity, conformity means that the data must adhere to a specified format, whether the country or the business dictates it. Returning to the date of birth field, a healthcare facility in the United States may use the form 05/01/1999 to represent a birthdate of May 1, 1999. On the other hand, a facility in Canada may use 01/05/1999 to represent the same birthdate. However, the data wouldn’t be valid if the entry read 01/05/1999 for a record in the United States, as this would mean that the patient was born on January 5, 1999.
An example where the organization dictates the standard for the data is the patient’s weight. Do they use whole integers or the decimal format? If using the decimal format, how many places do you go (e.g., 208.1 vs. 208.15)?
5. Uniqueness
Uniqueness means there are no duplicate entries in the data. For instance, a record for “Michael A. Johnson” and another for “Michael Arthur Johnson” may be referring to the same patient. These duplicates can cause data to drift if healthcare personnel update the two records at different periods.
6. Timeliness
The last metric to check is timeliness. As the name implies, timeliness means that the data is available when you need it. For example, a nurse that is about to attend to a patient consults the electronic chart for the medication type and dosage. That information should be readily available to them. As a patient checks in at a local doctor’s office, the receptionist should be able to pull up their insurance information quickly.
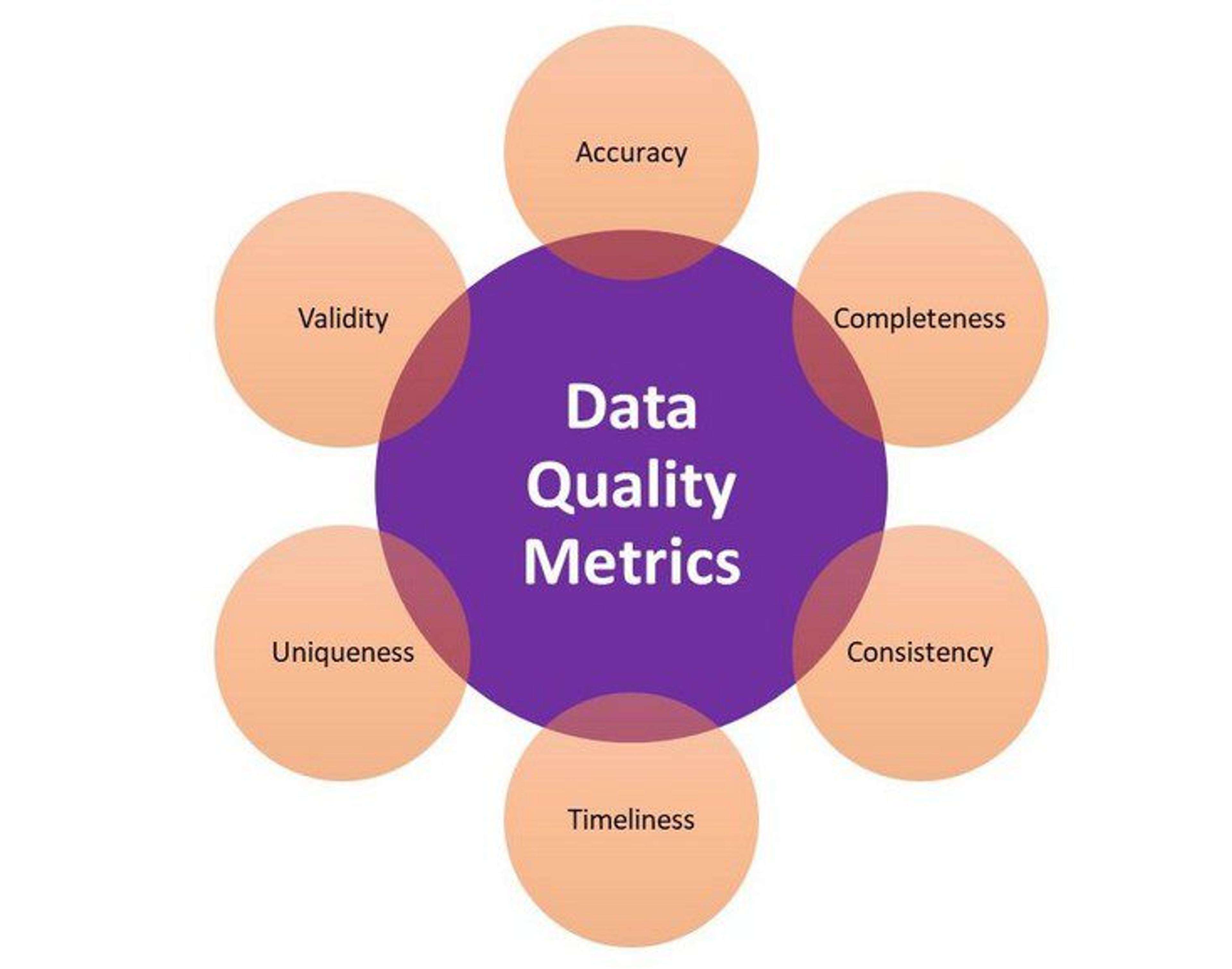
Image source: firsteigen.com
Leverage Sanator to Manage Your Your Data Quality Checks
Superior data quality translates to an improved patient experience, and Gaine’s Provider Network Management Solution, Sanator, provides all the tools to help you meet that goal in a single place. While other solutions are broad in scope to fit many industries, Gaine’s master data management (MDM) tool is focused solely on the needs of the healthcare and life sciences industry.
Contact us today to learn how Gaine can assist you in your data quality needs.